In our modern day, it’s difficult to find a sphere that hasn’t yet been touched by some representation of artificial intelligence. So, it comes as no surprise that we see a lot of applications of AI and machine learning in finance. The world of finance is heavily connected to numbers and calculations, which is something AI algorithms excel at. As opposed to other industries where human intuition and decision-making are vital to make accurate conclusions, financial analysis and predictions rely more on factual data. That’s why we can expect to see AI and machine learning remain an integral part of the finance industry. To understand the value of AI in the world of finance for the present and future, we’ll dive deeper into machine learning in finance from theory to practice.
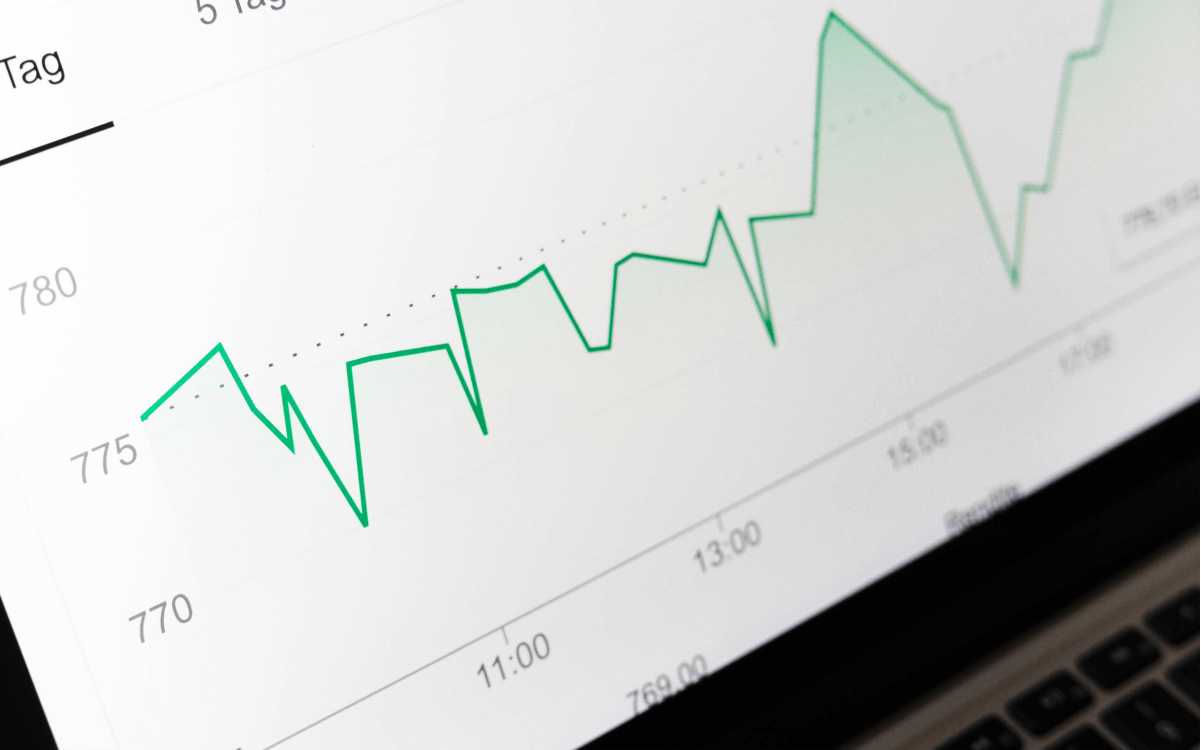
In this article, we’ll take look at all the latest coverage on the following points:
- Machine learning in finance
- Computer vision for risk assessment
- Advantages & limitations
- Key takeaways
Machine learning in finance
The presence of AI, computer vision, and machine learning in finance is not a new occurrence, but it is significantly developing to allow new prospects as we speak. Before we take a closer look at specific machine learning use cases in finance, it’s important to understand how computer vision at its core creates value for the industry.
By essentially allowing hardware to “see” and analyze the world the same way a person’s mind and eyes do, we can allow computer vision applications to execute tasks that would otherwise require a person to do manually. That is all thanks to the main computer vision tasks such as object detection (used for facial recognition software for authorization), optical character recognition (for scanning through text and documents), pattern recognition (for detecting anomalies in financial data), and more.
To get a well-rounded view of everything, let’s take a closer look at a handful of current machine learning applications in finance.

Underwriting
Loan underwriters are tasked with assessing applicants’ financing standings in order to determine if they will be able to take on a large loan or mortgage, how much of one, and how risky they are in the eyes of lenders. In order to come to such a conclusion, financial experts need to analyze dozens of documents before approving or denying a person a loan. That includes, but isn’t limited to analysis of their credit history, current income, debt-to-income ratio, and savings. AI models can be trained to assess that information and define whether the data falls within the boundaries of what is needed to qualify or be denied a loan. This can offer banks or credit unions an opportunity to go through significantly more applications and automate categorizing applications. Instead, the underwriters can review the assessments to ensure the human touch in the process is not lost and any discrepancies are accounted for.
Cashless & cardless operations
Technological advancements are paving the way for seamless shopping experiences. The move from cash purchases to credit cards was one step in the right direction and that is now followed up by transitioning to a world of fully digital purchases. Quick transactions via smartphones and smartwatches are already making headway and are predicted to only become the norm in the near future. They often operate with biometric recognition to use an individual’s fingerprint, iris, or face to authorize payment and that is possible to achieve with our accurate, modern-day facial recognition software.
Fraud detection
It is inevitable that fraudsters and hackers are attracted to the world of finance. For that reason, a bank or other institution needs a strong shield to protect themselves, and their clients, from fraud and deception. That ranges from stealing a credit card and attempting to steal money from it all the way to bypassing the digital firewalls of banks to commit fraud. Machine learning applications are able to tackle those issues by identifying them before they wreak havoc. For example, face recognition software installed on ATMs helps track illegal credit card activity. Additionally, complex algorithms can detect changes in the data to determine when and if the institution's records have been tampered with.
Computer vision for risk assessment
Yet another vital way machine learning and finance are connected is by carrying out elaborate risk assessment. All of the machine learning use cases in finance we mentioned above are all viable, but there is something that banks and financial institutions need more than anything — low risks, and higher guarantees. Whenever a new client opens a credit card or opts for a loan, the bank is entrusting borrowers with financing that has the possibility of being left unpaid. If the outgoing proceeds outweigh the profit turnaround, then banks are left in risky circumstances.

With that in mind, AI and machine learning for risk management is becoming a major asset for the finance industry. Computer vision is not only resourceful when it comes to analyzing existing data, but it goes forward another step to utilize diverse data, recognize patterns, and generate data-driven predictions. Those predictions can go on to mitigate financial risk for a bank or credit union. You may be wondering exactly how to manage financial risks with machine learning. AI can be entrusted with processes such as carrying stress tests among loan applicants, detecting early warning signs of risks based on industry trends, screening for fraudulent financial data or discrepancies, reviews of portfolio structure, and so on.
Advantages & limitations
For the time being, the volume of machine learning applications in finance an institution decides to take on is up to them. It’s necessary to do a cost-benefit analysis to determine that the advantages of implementing AI applications will outweigh the limitations. Let’s shed some light on the advantages and limitations of machine learning in finance.

Advantages
- Decrease in manual processes — The biggest advantage for AI across the board is taking over tasks that are otherwise performed manually by people. This is often confused with utterly limiting the need for human employees in institutions when in reality, that is not the case. Computer vision allows AI to handle tasks that would otherwise tediously take up hours to days of a financial analyst’s or banker’s time, allowing them to concentrate their efforts on matters that absolutely require their attention. Looking at the process of accepting a credit card application and opening an account for an individual, for example, we’ll see that the institution needs to carry out several steps to execute this request where appropriately implemented AI can automate the process.
- Streamlined and quick approach — Aside from merely decreasing the workload from the bank’s perspective, clients of a bank or other financial institution can expect quicker processing times and turnaround related to their inquiries. Processing times on tasks such as credit card and loan applications can be cut down in the case of automation. Customer service will become fast-paced with the help of advanced bots and can be provided on a 24/7 basis. Finally, clients can opt for AI-driven financial consultations tailored to their schedules and preferences.
- Accurate, fact-driven predictions — Utilizing machine learning for financial predictions and risk assessments is driven by factual data, which also decreases the margin of human error in such processes. All of the predictions are made after assessing the available training data, making conclusions accordingly, finding gaps, and offering data-driven predictions. This will help considerably in detecting fraudulent behavior that is occurring immediately among the members of a financial institution.
Limitations
- Customer-institution challenges — Regular clientele of banks and financial institutions are used to the traditional way of things which often involves paper-and-pen processes and face-to-face communications. It is difficult to expect customers to quickly become acquainted with new ways of doing tasks that they’ve done before. For example, clients may not have much trust and faith in algorithms calculating their eligibility for applying for a credit card or a loan. Additionally, business owners may not like receiving financial consultations from AI-powered robots, and so on. Filling the gap between consumers’ attitudes towards AI-forward finances and the institutions’ needs for innovation will require a gradual approach.
- Cost of acquisition and implementation — Not all applications of AI are available for mass consumption at the moment. Typically, for the most customized approach to problem-solving, companies opt to have in-house data science teams to construct custom ML or DL models. The other option is tasking a third-party company with creating a custom pipeline. Both are costly investments though. Since AI software (and the necessary hardware) are not easy to acquire at the moment by everyone, financial institutions will be off-put by AI implementation until it is more accessible.
Key takeaways
Today we answered the all-important question of how machine learning can be used in finance along with taking a look at computer vision applications in financial risk management. AI is allowing clients and banks to have seamless and secure experiences, along with paving the path for future innovation. Computer vision in financial risk assessment in particular is a great asset for banks and financial institutions to help receive an automated and data-driven overview of their liabilities and risks to aid with mitigation. Since the advantages of AI in financial institutions outweigh the cons, its implementation in the industry is expected to become the norm.
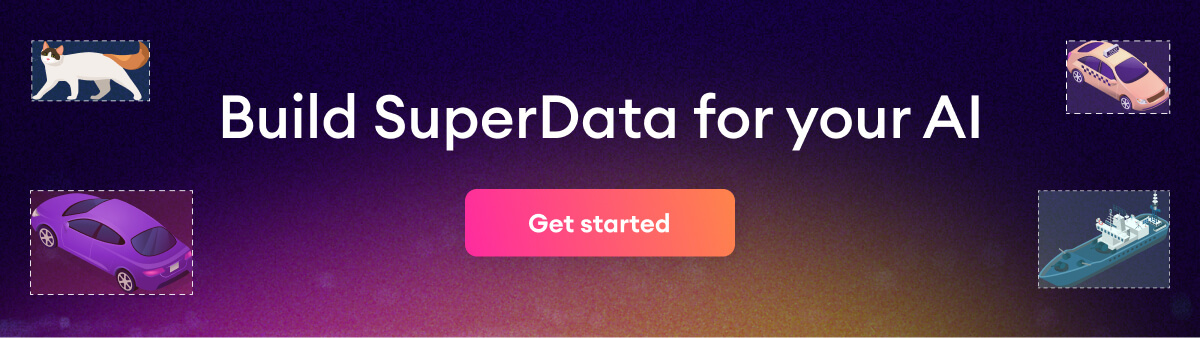