Taranis, a precision agriculture company, wanted to transform complex drone and satellite imagery into clear, actionable agronomic recommendations using generative AI. Their vision was to automate the analysis of millions of images to provide concise, intuitive insights for farmers.
However, their initial methods—relying on prompt engineering and spreadsheet-based annotation—faced challenges with scalability, speed, and accuracy. To meet a critical seasonal deadline, Taranis partnered with SuperAnnotate to optimize their annotation workflows and efficiently fine-tune a specialized AI model.
Within just six months, Taranis successfully deployed a robust, highly accurate GenAI assistant, empowering farmers with quicker and better-informed decisions.
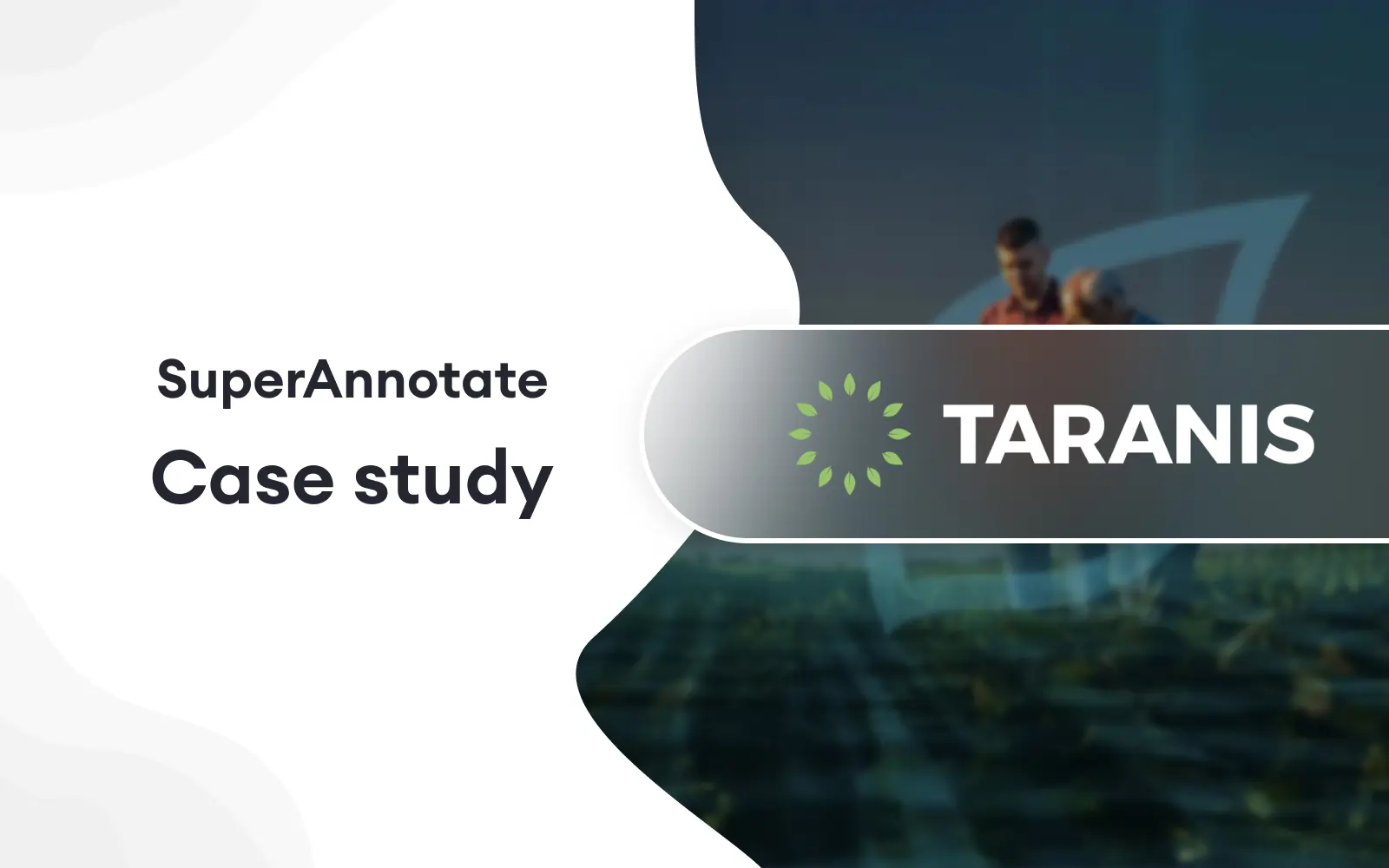
The challenge
Developing an effective agronomic AI assistant was complicated. It required sophisticated reasoning, combining factors such as crop health, weather patterns, planting timelines, weed management, and disease detection.
Generic large language models struggled to handle this complexity:
"Prompt engineering only got us to about 50% acceptable outputs. The logic was too complex."
— Gershom Kutliroff, CTO, Taranis
Additionally, Taranis initially relied on manual annotation processes using Google Sheets, leading to fragmented reviews, slow iteration cycles, and developer-dependent UI changes. With the planting season rapidly approaching, Taranis urgently needed a faster and more efficient solution.
The solution
Taranis turned to SuperAnnotate to streamline their data annotation and model training pipeline, significantly accelerating the entire process.
Key benefits of the collaboration included:
- Custom, no-code annotation UI: Agronomists easily built specialized interfaces to review AI-generated summaries, tag inaccuracies, and identify logical errors—without developer assistance.
"Other platforms required front-end developers just to get started. With SuperAnnotate, I could do it all myself."
— Itamar Levi, Lead Agronomist, Taranis
- Rapid iteration cycles: Centralizing annotations and reviews reduced turnaround times for prompt adjustments, quality control, and model retraining.
- Real-time collaboration: SuperAnnotate's platform enabled seamless teamwork among agronomists, data scientists, and QA, resulting in quicker decisions and faster progress.
Results and impact
SuperAnnotate's streamlined workflow and collaborative tools significantly accelerated Taranis's model development, delivering measurable improvements across the board:
- Model accuracy increased to 95%, dramatically improving from under 50% previously.
- Annotation review time reduced by 80%, dropping from over 10 minutes per annotation to just 2–3 minutes.
- Dataset size expanded by 50x, enabling large-scale fine-tuning and continuous improvement.
- Saved the equivalent of 1 full-time position by empowering domain experts to manage UI adjustments directly.
- Completed the entire project in just 6 months, meeting the crucial spring planting season deadline.
“Without SuperAnnotate, we would’ve had to release the product with a limited feature set. It made a huge difference to our ability to launch the full product on time.”
— Gershom Kutliroff, CTO, Taranis
What's next
Building on this success, Taranis plans to incorporate reinforcement learning from human feedback (RLHF) to further enhance the clarity, accuracy, and effectiveness of their AI-generated recommendations.
About Taranis
Taranis helps farmers optimize their decisions through advanced data analysis from drone imagery, satellite data, and field observations. Their platform provides comprehensive solutions for crop monitoring, weed detection, and disease management across millions of acres.
About SuperAnnotate
SuperAnnotate is an end-to-end platform for building robust AI data pipelines. It offers highly customizable annotation interfaces, integrated quality assurance, and versatile support for multimodal data, enabling teams to accelerate AI development.
Ready to accelerate your GenAI projects?
Discover how SuperAnnotate can help you build better datasets and achieve faster model deployment.
