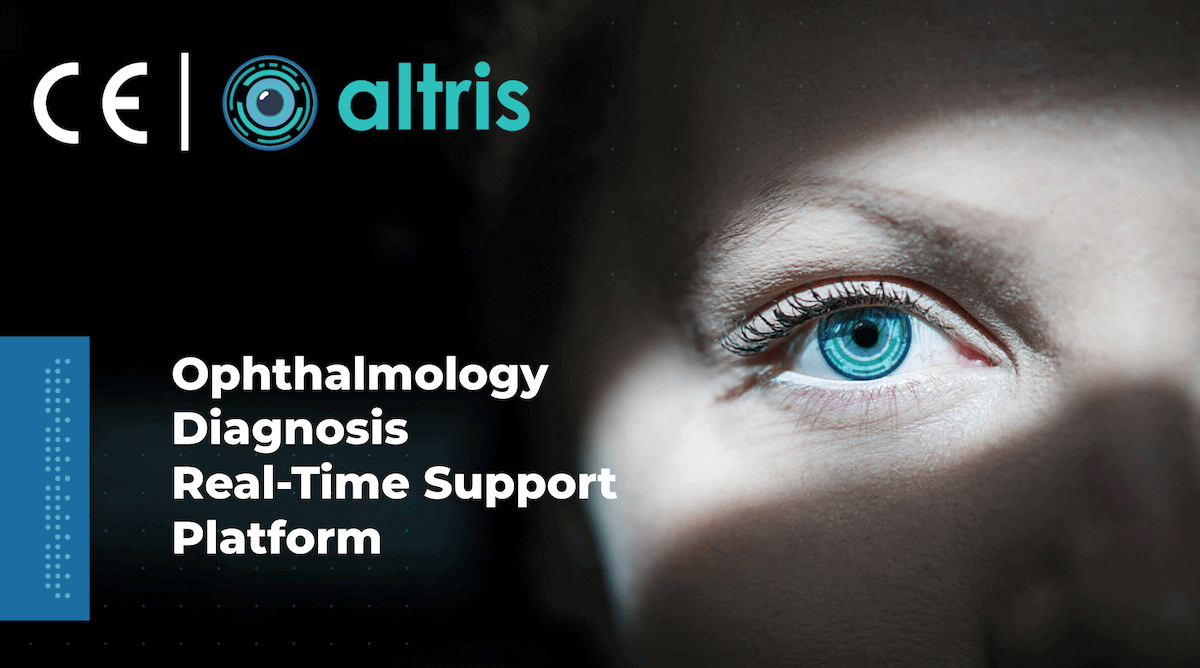
Company overview
Altris AI applies computer vision and deep learning algorithms to build real-time innovative ophthalmology diagnosis support for automatic, structural, and quantitative analysis as well as detection of retinal diseases on Optical Coherence Tomography (OCT) scans

How Altris AI uses SuperAnnotate
Altris AI uses SuperAnnotate to manage their in-house annotators who are ophthalmologists with extensive expertise in ophthalmic retina imaging.
Previously at Altris AI, we used Labelbox for the segmentation of pathological areas on OCT scans, and AWS Sagemaker for OCT scans classification. We weren’t happy with the annotation quality of Labelbox due to the poor usability and accuracy of their annotation tooling, and we noticed low-quality annotations in pathological areas. As a result, we decided to search for alternatives.
After a long search, we selected SuperAnnotate due to the platform’s significantly more robust toolset, superior ease of use, and more powerful project management features. As mentioned before, our annotators are medical doctors, not professional annotators. We therefore, needed a tool that had super-easy usability and a great user experience and SuperAnnotate was that platform.
In addition, thanks to powerful analytics, they can also measure the performance of each annotator and solve performance issues. These metrics help our team at Altris AI manage their annotators more effectively.
SuperAnnotate vs Labelbox
We used both tools extensively. And while there were a number of differences between the platform, there are 2 key differences I want to highlight:
User Experience - SuperAnnotate’s user experience is vastly superior. Simple features like scrolling and zooming, all the way to complex features like shortcuts and QA were much more convenient, easier to use, and more robust in SuperAnnotate than in Labelbox We had trouble in Labelbox with things like difficulty with navigation, workflows, and pixelation of images when zooming in that made it difficult and time-consuming for Altris AI’s medical doctors to annotate pathological structures correctly.
Tooling - SuperAnnotate’s polygon tool is much faster, more accurate, and more robust than Labelbox. Pathological structures can have different sizes and configurations, and small pathological structures can be found in larger ones, making the polygon tool an extremely useful feature. Users can create polygons inside larger polygons using SuperAnnotate. However, that’s not the case with Labelbox where polygons cannot be created when lines touch one another. In addition, tasks like drawing many-pointed polygons and editing existing polygons were all much faster and easier in SuperAnnotate.
Key results
- Switching from Labelbox to SuperAnnotate resulted in significant improvements to our annotation practice. Some key improvements were:
- Model accuracy saw an increase from 80.1% to 92.4%.
- In addition to improved model accuracy, annotation time went down (despite annotations being more precise and complex) from 5.8 min/scan to 4.9 min/scan, which is a huge performance increase with our volumes of datasets.
- QA time was cut in half from 2 min/scan to 1 min/scan.
Summary
- SuperAnnotate decreased our annotation cost and drastically increased our annotation quality.
- SuperAnnotate boosted model performance by almost 15% while reducing annotation time, and cutting QA time by 50%.
- SuperAnnotate provides excellent customer success and support. It’s always easy to stay in touch with their team and they’re very responsive
Author: Andrey Kuropyatnyk, CEO at Altris AI