Everyone can enjoy the taste of a juicy hand-picked apple from the orchard. What if the whole process, from sowing to harvesting, was completely supervised by a robot without resorting to a single human? Well, computer vision technology is developing at an accelerated rate, making its way into many (nearly all) industries, and agriculture is no exception. With the growing population and food demand, we're going to see the rise of agricultural automation, with the help of computer vision, become a regular occurrence sooner rather than later. Let's dive in further to understand what are some new avenues for innovation in computer vision in agriculture entails.
Here's a closer look at what we'll cover:
- A quick overview of computer vision
- Computer vision in agriculture
- Aerial imaging and scanning
- Key takeaways
A quick overview of computer vision
Computer vision is a branch of artificial intelligence that allows machines to possess the capability of thinking and interpreting visual data as a human would. Computer vision systems analyze an array of visuals from images to real-time footage and can track, label, describe, predict, and assess particular objects within those visuals. Its aim, especially with the incorporation of deep learning, is to train artificial intelligence to the point of going beyond simply automating processes. For example, when we look at a scene unfolding in front of us, our eyes make a note of what objects we see, where they are located, where those objects move to (if they move at all), what we can potentially predict happening, and so on. Computer vision technology can incorporate all of that by training artificial intelligence based on the existence and pattern recognition.
With the way things are unfolding now, specialists expect to see significant development and spread of computer vision applications that will change the modern world as we know it. For example, the notion of shopping may be revolutionized sooner than we think by shifting to cashier-less stores. All of that is possible to a foundational component of computer vision called semantic segmentation — the technique of assigning a class label to each individual pixel in an image.
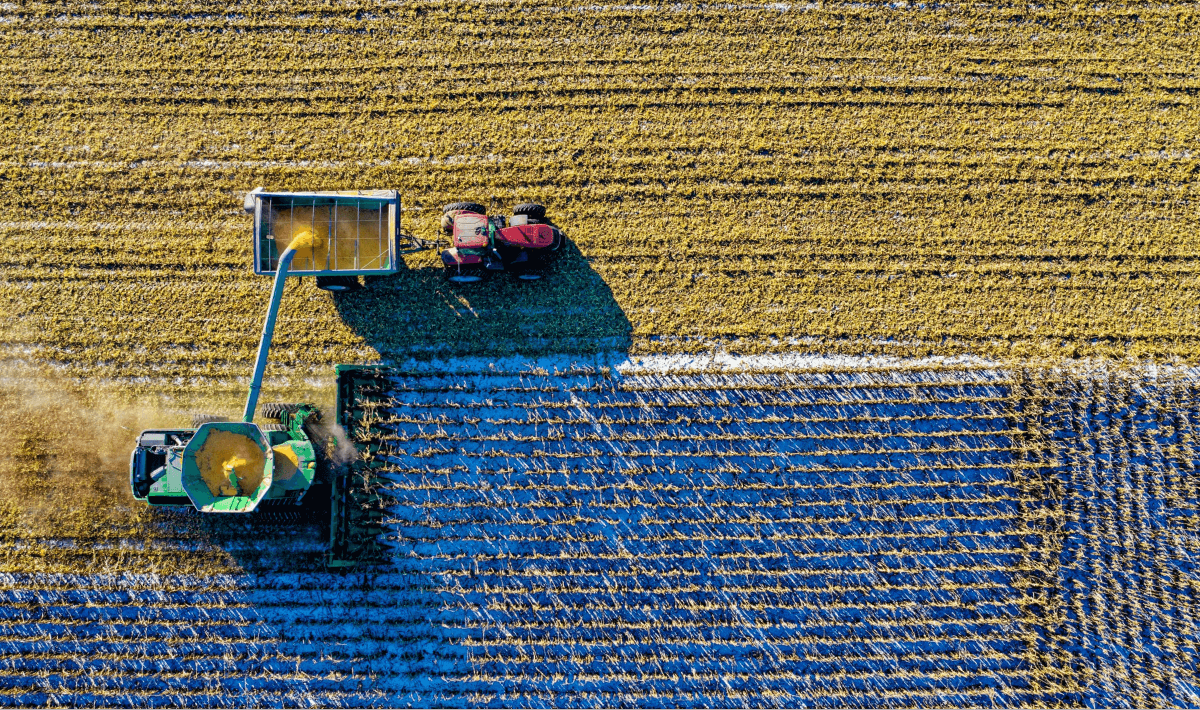
Computer vision in agriculture
Agriculture, livestock farming, and agronomy are no exceptions to the widespread surge of computer vision techniques. As one of the oldest occupations known to man, farming and agriculture are still being advanced when mixed with new computer vision and machine learning technologies. Adapting deep learning and machine learning applications in agriculture is a game-changer since it gives more efficient, accurate, and automated processes within the industry, putting us one step closer to the future. With computer vision systems and the vast amount of data it provides, it is no surprise that farmers now get to make better-informed decisions and come up with faster solutions. Computer vision applications in agriculture, such as detection and classification, combined with state-of-the-art hardware, bring such technologies out of the screen and into the field.
Now that we have covered the introduction to computer vision in agriculture, let's take a look at specific uses of computer vision in agriculture.
Harvesting with machines
It is safe to say that the advantages of computer vision agriculture are numerous, which means that agricultural automation-fundamentals and practices can vary. Machines are a time-saving and efficient way to harvest crops, but we aren't talking about regular farming machines like harvester combines; specialized robots are equipped with object recognition and deep learning technologies to harvest fruit and vegetables autonomously. Now farmers get to sort and recognize their crops through machine vision systems, and specialized machine learning models will identify the condition of the crop. That is made possible with the combination of two components: First, the act of grasping, which is difficult and is done by specialized hardware, and second, the software or visual part, which identifies the objects to ensure proper grasping.
- Benefits: The implementation of machine vision in agriculture significantly accelerates process times and reduces the need for manual labor. The latter is especially beneficial during harvest seasons when fruits and vegetables are left unattended and decayed on the ground.
- Challenges: Fruit detection is one of the most difficult tasks in agricultural automation. The complications are a result of unpredictable variables in the process that can skew results, such as inconsistent illumination, poor visibility, or occlusion due to foliage, not to mention inconsistencies in the shape of the fruit or vegetable.

Autonomous tractors
Similar to autonomous vehicles, autonomous tractors are becoming a big hit in the agricultural sector, relying on computer vision and cameras to get a full 360-degree view of their surroundings. When using autonomous tractors, time-consuming tasks such as harvesting and plant removal can be done in a faster and more sustainable manner. These tractors need the help of neural network algorithms so they can analyze the data they captured through their cameras, and later use it to fixate their algorithms and improve performance.
- Benefits: With computer vision, tractors can now tag crops, allowing the farmers to see the changes in plants while also tracking necessary trends that develop crop yield. Plows and tillers that have computer vision systems come with their own trailer, and that makes the whole process automated, categorizing soil conditions and estimating when it needs seeds.
- Challenges: Other than the fact that it is a large investment, there is also the aspect of legal accountability when it comes to acquiring autonomous tractors. As autonomous tractors will be operating in public areas, there’s always the risk of a collision, hurting the soil and plants, or even injuring humans and animals.
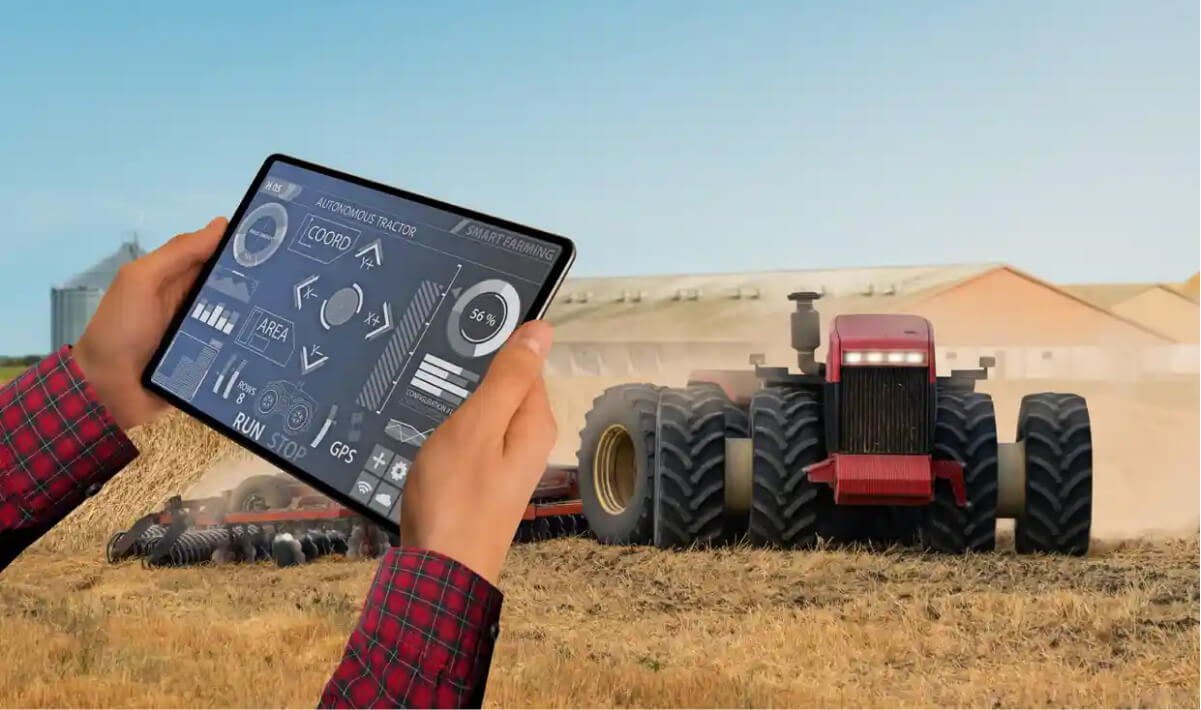
Grading and sorting
Assessing features such as ripeness, color, size, and defects is a task that pomologists and other agriculture workers carry out quite often for quality assurance of their harvest. Furthermore, they assess the needs of a particular crop, such as whether it needs more water or different antibiotic doses. What if we told you there is ready-made technology out of the visual world that deals with the scanning and labeling of fruit and vegetables in real-time?
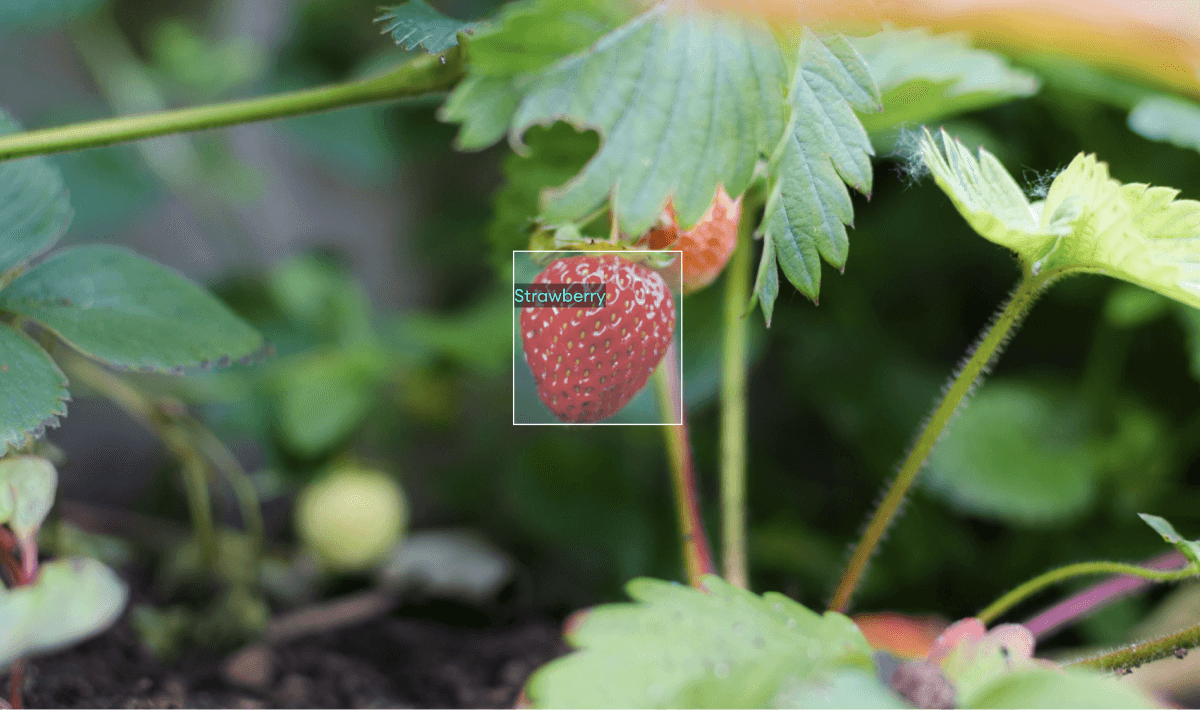
- Benefits: Traditional grading and sorting are human-dependent, labor-intensive, and take up significant amounts of time. For larger farms, more manpower and manufacturers are required so that they get to sort and grade hundreds of thousands of produce each day. That is where computer vision comes in to innovate the process via scanners equipped with image classification technology. By utilizing artificial intelligence and computer vision algorithms, agriculture workers get real-time monitoring of crop growth and satellite imaging of their conditions.
- Challenges: Initial technology and updated prototypes yield promising results but need to be optimized for a number of specific shortcomings. Firstly, scanning of the produce via a 2D image may result in inconsistencies if the produce, for example, a tomato, has an apparent marking on the other side that was left unscanned. Additionally, the biological variation among the fruits and vegetables must be considered to yield accurate results.
Vertical Farming
Vertical farming is revolutionizing traditional farming by growing crops indoors, vertically, amplifying crop output in a small space, rather than letting it depend solely on land, machinery and water. Vertical farming amplifies yield and space, as it uses computer vision to observe a plant’s lifecycle through its cameras and sensors.
- Benefits: Making the most out of recent agriculture technologies, vertical farming can control the temperature, gases and lightning. It also reduces water usage, cuts down on labor costs, guarantees a more persistent crop production, and uses energy more efficiently.
- Challenges: As vertical farming takes place in indoor environments, it doesn’t allow insects to get in, affecting the process of pollination. When reverting to vertical farming, farmers need to consider such aspects and resort to manual pollination. Another downside to this method is that it is very much technology dependent, meaning that the smallest issue with either the lightning or temperature could affect the whole process.
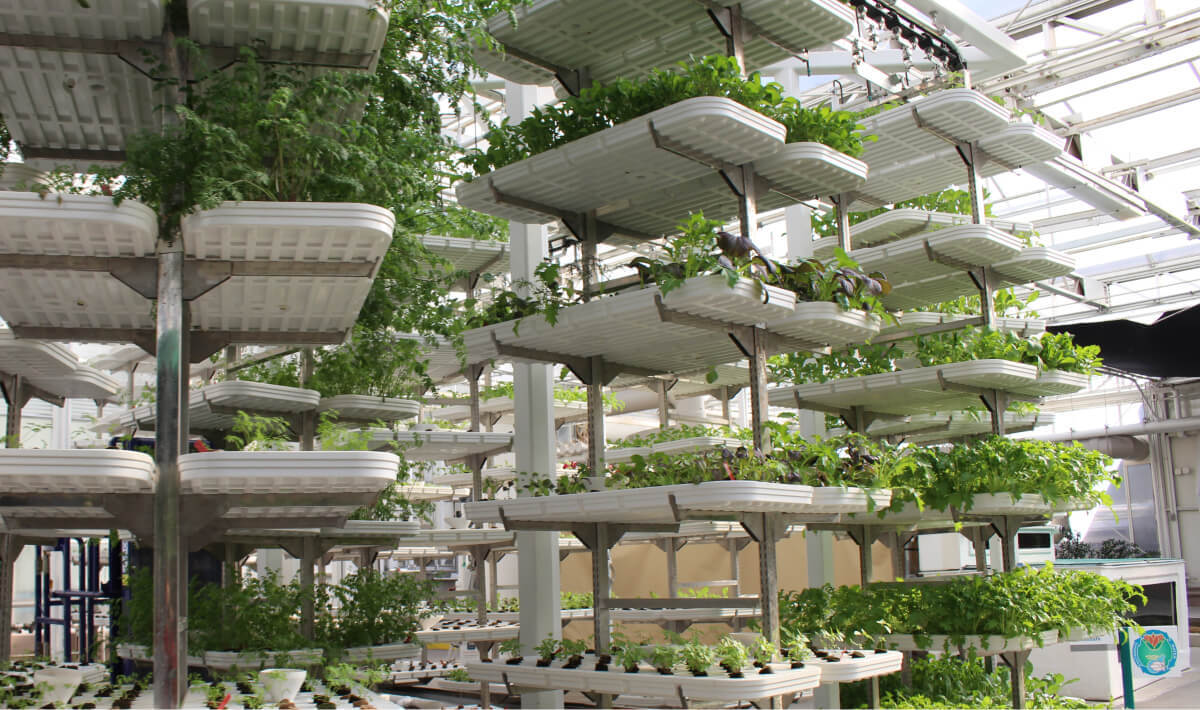
Aerial imaging and scanning
Unmanned aerial vehicles (UAVs) are becoming widely utilized in recent years for tasks such as remote sensing and fire detection. There's no stopping there since aerial imaging and scanning with the help of computer vision technology aims to establish what will be known as “farming vision”. The use of UAVs specifically aims to be an asset for precision agriculture, otherwise referred to as aerial agriculture. Precision agriculture aims to boost eco-friendliness, yield estimation, and efficiency, all while reducing the amount of traditional input required to cultivate crops such as land, water, fertilizer, herbicides, and insecticides. That can be possible through precise aerial precision imaging and mapping to assess each region based on the results of the imaging.
- Benefits: UAVs equipped with extensively trained computer vision models are the key to smart farming as they carry out processes such as vegetation classification and segmentation, crop counting, yield monitoring and prediction, crop mapping, weed identification, and disease and nutrient shortage detection. Essentially, they allow the people in the agriculture sector e.g. farmers and field workers, to engage with crops remotely and only interfere when the data acquired from the UAVs deem necessary.
- Challenges: Despite all the above-mentioned benefits, there are still limitations to be addressed with computer vision in aerial agriculture. That includes the nuances of plant dispositions across a set period of time. For example, different plants go through different phases throughout the seasons, at different times, for a variety of intervals. The technology must be foolproof in its deep learning capabilities to assess these spatial changes without compromising accuracy.
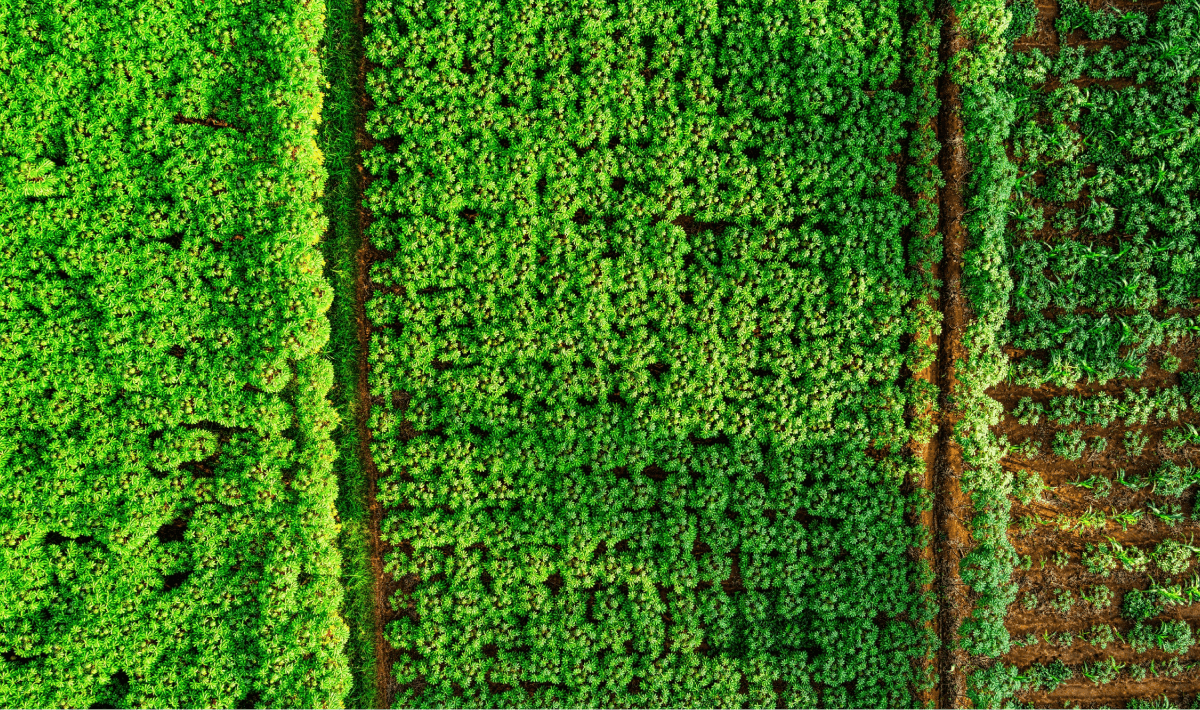
Plant phenotyping
Plant phenotyping is a task that falls under precision agriculture that involves the systematic evaluation of plant characteristics such as growth, development, adaptability, quality, tolerance, resistance, and structure. It is similar to the computer vision technology used for grading and sorting however it expands to help the research of botanists as well.
- Benefits: Once again, this form of agricultural automation projects can minimize the manual labor required by specialists in the field to analyze each plant one by one. With the help of such technologies, those working in the agricultural sector can quickly acquire the data, assess the annotated information, and then take samples of plants they believe have diseases to the lab for testing.
- Challenges: While computer vision models showcase great prospects for accuracy, detection, and automation, this mass of data still requires significant input by scientists. The AI must be trained to account for weather changes, plant species variations, lighting changes, and an entire plethora of diverse scientific data required to assess the plants accurately. It is still a task that requires the supervision and investment of specialists before the technology can be fully self-sufficient.
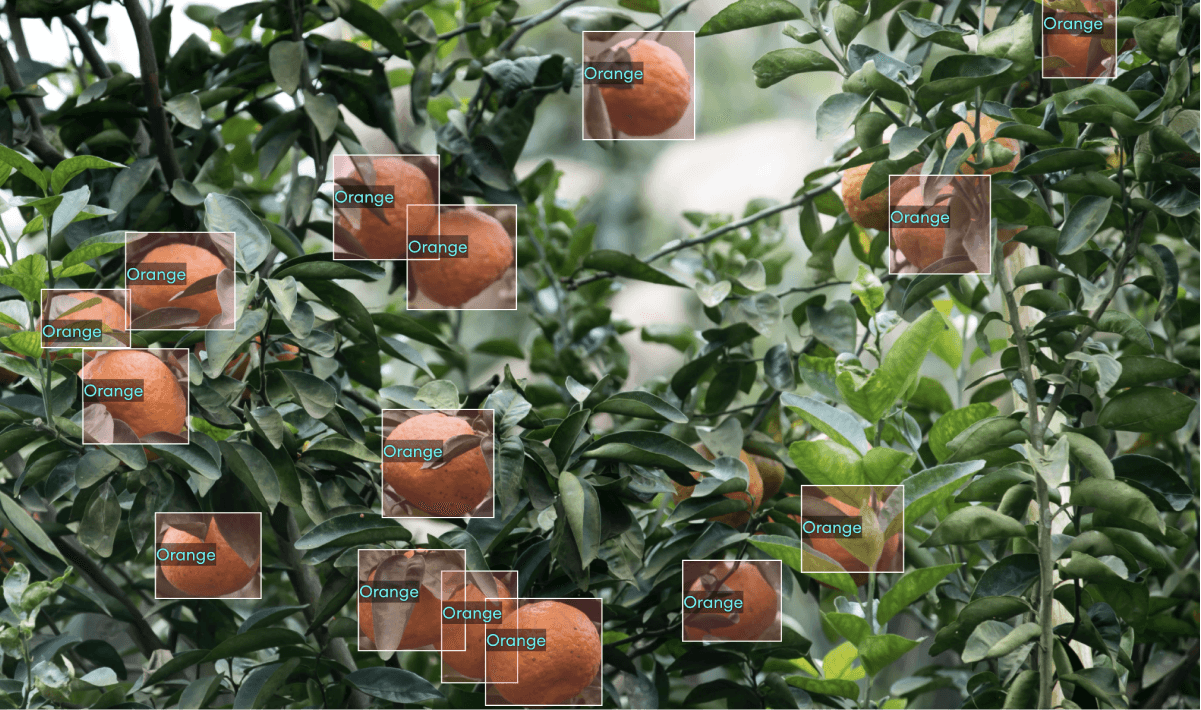
Key takeaways
Overall, the impact of computer vision systems and deep learning methods in the agriculture sector is on a positive incline since there is more progress being made than setbacks. The preexisting and currently developing applications of computer vision in agtech cut down on manual labor in the field, increasing the speed and accuracy of many operations. Along with the spread of agriculture machine learning data, agricultural robotics, and automation, the industry can now solve some of its biggest issues including sustainability, climate, and labor shortage. With such technological advancements in agriculture, the industry does not need to be associated with long hours in the field conducting grading and scanning of fruits and vegetables by hand or straining physical health anymore. It became clear that automation best solves agricultural shortages, and with that, crops may be tended remotely, harvests collected effectively, and mass production of goods will be accelerated, all thanks to artificial intelligence. With technology and agriculture going hand-in-hand now, farmers, pomologists, botanists, and many more workers in the agricultural sector can dedicate their time to other processes in their field of work. Their presence in the field won't become redundant with the rise of artificial intelligence in agriculture since they are essential for the process of training datasets and assessing anomalies in data acquired via machine learning or deep learning technology. Tools are at the foundation of agriculture, and with computer vision, those tools can only become more enhanced and take computer farming to the next level on an international scale.
SuperAnnotate helps companies annotate, label, and utilize higher-quality data. If you’re looking for a reliable tool to accelerate in your field, you came to the right place. Schedule a call, and we'll be more than happy to answer all of your questions.
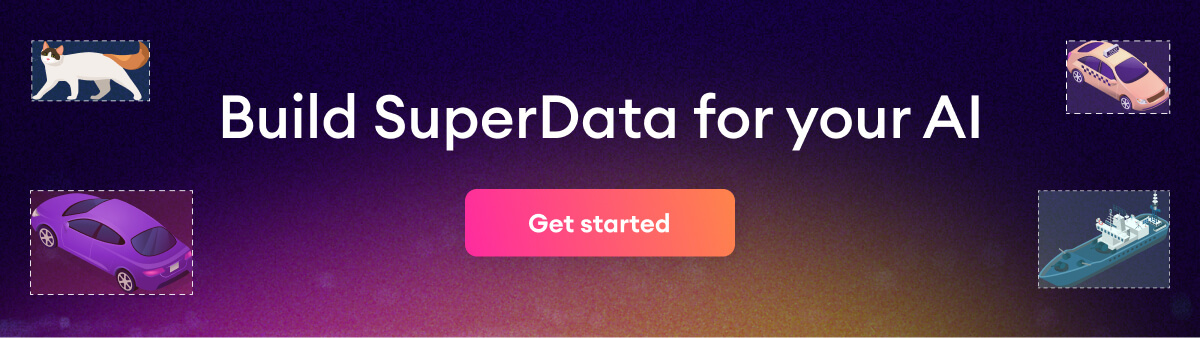