We all have been there: clothes scattered all over the place, shelves half-empty, and soon enough, you find yourself stepping on dirty piles of laundry. Plenty of outfit options are within a hand’s reach, but you need the exact shirt, pair of pants, or the tie you’ve imagined yourself in for quite a while. On top, you’re ridiculously late, and you’re on the brink of missing your daughter’s birthday, friend’s wedding, or, worst case, your own elevator pitch. Now, the lesson learned: no matter where you go or the field you operate in, your data — just as your clothes — need organization. One cannot overstate the importance of data management for a business, and this is where it becomes essential to understand the differences between data lakes, data warehouses, and data swamps. Without further ado, let’s jump right in with the following breakdown:
- Data lake vs. data swamp
- Why do you need a data lake?
- Data lake benefits and drawbacks
- How to avoid a data swamp?
- Data warehouse: Types and applications
- Introducing the data lakehouse
- Main takeaways
Data lake vs. data swamp
The term data lake has sparked controversy and misuse in the beginning, challenging the traditional data storage architecture. As irony would have it, any data structure could be referred to as a data lake at the beginning, which is understandable as water-related metaphors were and still are abundant in data science. With time, this freewheeling concept has slowly grasped common context. The emergence of a data swamp, as a science term, has had a similar path. Though data lakes and data swamps have profound differences these days:
The overarching similarity between data swamps and data lakes is that both represent data storage or repositories. Data swamps usually begin as a lake, ending up in a swamp only when businesses, companies, or data owners do not set up concrete expectations and guidelines for their data repository. Soon enough, they find their data filled with unrelated files and fluff, which makes it a challenge to search for concrete items in unorganized storage. One of the typical characteristics of a swap is the lack of administration and governance, which makes it difficult to access and manage the data, and thus, poses a hazard for businesses that need to keep constant and precise records of data.
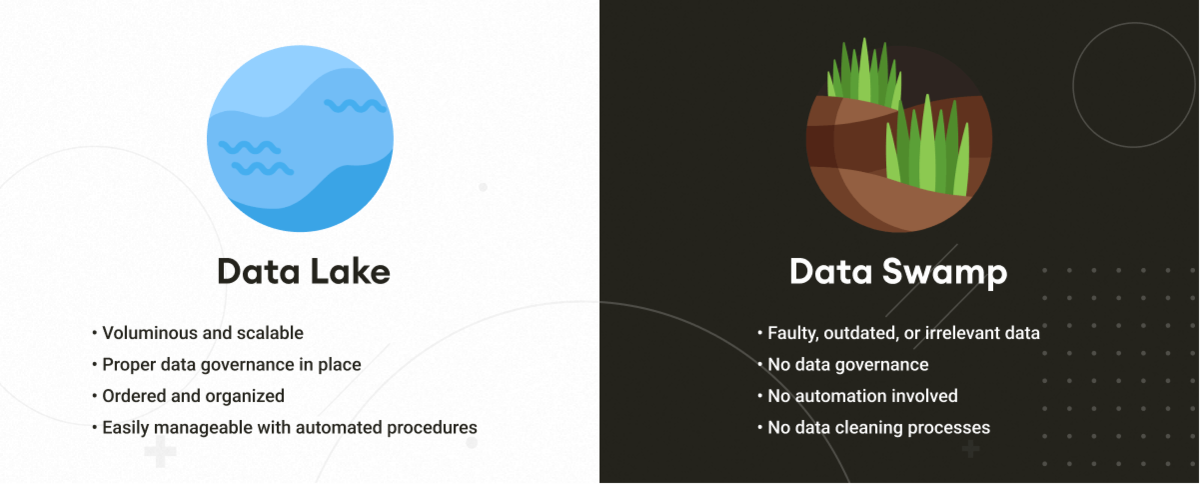
Why do you need a data lake?
When purposefully set up, with proper metadata, close governance, and intuitive organization, data lakes have the potential to become a valuable resource and insight for businesses. That requires initial commitment and a systematic approach to data management right at the outset.
Data lake benefits and drawbacks
Below we’ll cover some of the advantages and disadvantages of using data lakes.
Pros
- Volume and scalability: Data lakes are a free ride: you derive value from not just voluminous but multi-purpose data. Hence, one of the advantages of a data lake is the efficiency of the organization even with a plethora of information, which provides room for infinite scalability even with multiple data formats.
- Centralization of the data: Data lakes retain all data in the raw format: You have both data that you know you need to use, and data that you’re not sure how to handle yet. This opens up the possibility to store more data and thus, some machine learning and deep learning applications can be readily implemented here.
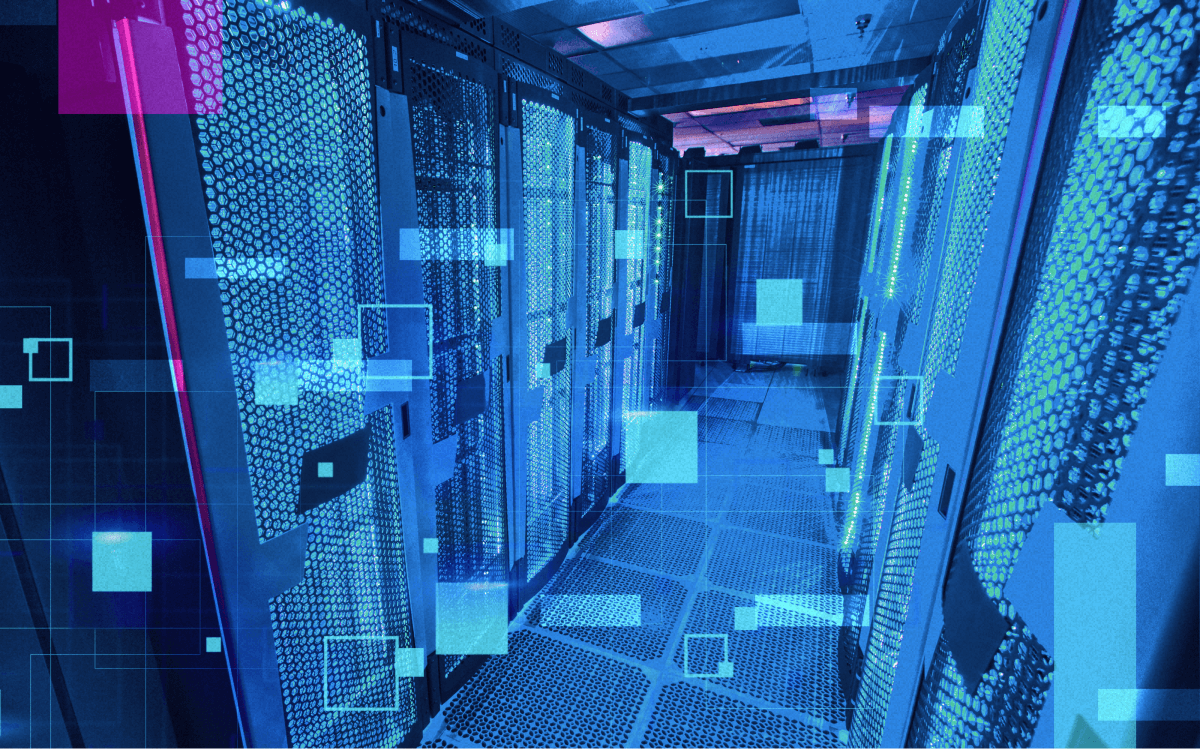
Cons
- Security and privacy: With massive volumes of data, privacy concerns are inevitable, albeit they define a usable lake. Imagine not having an upper hand when sensitive data lives in a lake and is, thus, at the risk of being exposed to anyone with access to the lake. To maintain a healthy data lake, it is imperative to navigate through role-based access control, encryption of data at rest and flowing free, authentication issues, and more throughout a data lifecycle.
- Regular maintenance: Face it, when you have loads of data stored in a lake, it is cumbersome and resource-intensive to extract analytics. Gliding through data lakes can be time-consuming, and despite the common belief that data lakes require little-to-no maintenance, without constant management and “launder,” a lake can — fairly enough — transform into a swamp. A proper data governance framework in place, nonetheless, can prevent a similar shift.
How to avoid a data swamp?
Data is the new oil: it is at the core of every major company’s success, and setback, so poor data management and navigation can cost you a fortune. Now, data swamps are basically lakes that encompass low-quality unstructured data, which is what you want to avoid. Here are a few steps to prevent a data lake from becoming a swamp:
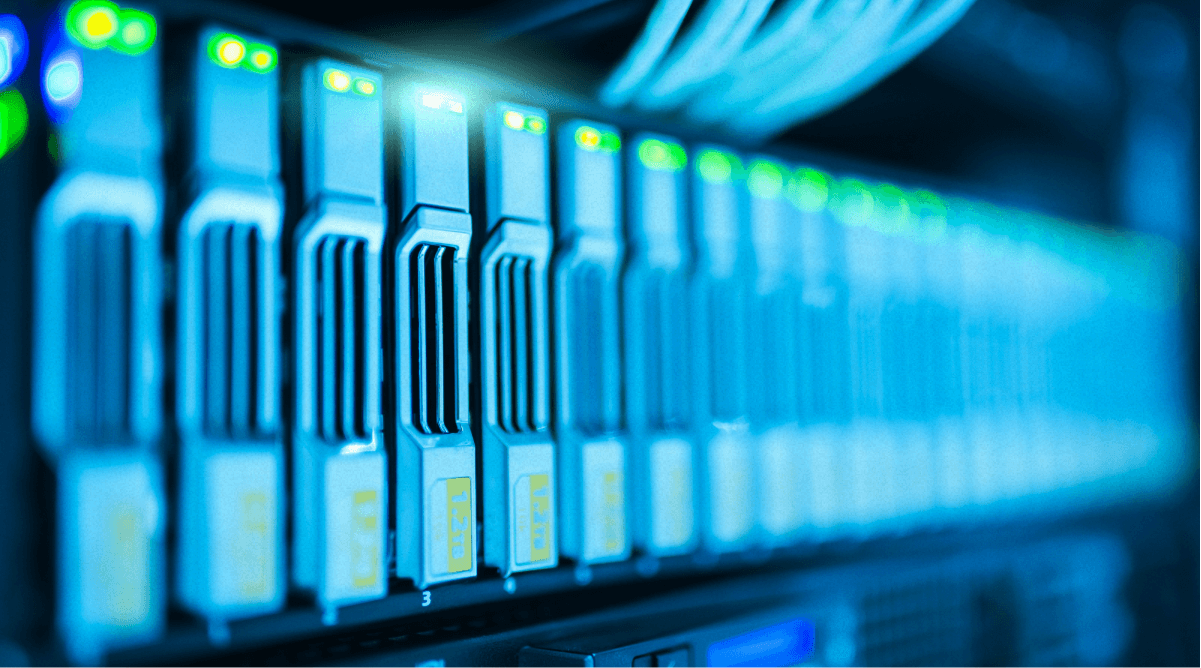
Plan the structure beforehand
You can store data of any type in a data lake, yet it all comes down to the balance of making your files structured but also open and inclusive, instead of just throwing things willy-nilly. The efficiency of your lake depends on the established hierarchy of the files or how well your dataset is organized, and how you keep managing your files.
However, don’t rush to get everything flowing free into your lake: If it is not organized right at the outset, it will become even more difficult to navigate through it in an ever-growing environment. You have to have a good sense of what’s where, and catalog your data on ingest to make it searchable.
Document changes made to the data in-store
Another common issue with data lakes is dumping the same data all over or integrating it with different datasets without being aware of whether or not the data has been integrated into another project. In short, with data lakes, there is always the risk of having different versions of the same data appear in a lake. Meanwhile, documenting the data lineage, the interactions people have had with it, and implementing a solid data governance framework could have saved a fair amount of time and effort keeping the data quality on track.
Data warehouse: Types and applications
If data lakes are vast data repositories, then you may wonder what’s the difference between a data lake and a warehouse? Data lakes emerged off of the necessity to manage big data to use raw and unstructured data to the fullest extent. Warehouses, on the contrary, were born out of the need for analytical queries for business executives to empower decision-making. So, modern cloud data warehouses are largely accessible to companies even with limited resources at hand and can be seamlessly integrated with business intelligence tools. This lays a solid foundation for a data-driven culture across companies, as warehouses grant the flexibility of access control, governed data, and more. Moving along, we’ll discuss three primary types of data warehouses:
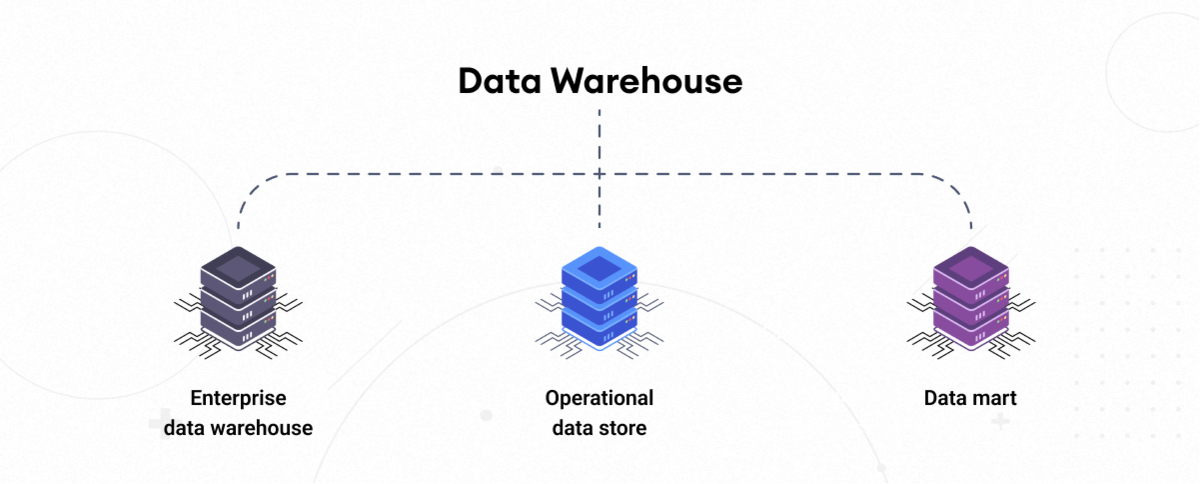
Enterprise data warehouse
An enterprise data warehouse, or EDW, is a collection of databases that centralizes business information for use in analytics and business processes. It can be housed on an organization's own server or in the cloud.
Operational data store
An operational data store, or ODS, is a central database that combines data from multiple sources into a single destination for reporting. This enables businesses to combine original data from various systems into a single place.
Data mart
A data mart is a smaller version of a data warehouse, designed to focus on a single subject or line of business. Quicker access to data and insights can be achieved through this structure. With data mart, teams don't have to surf larger warehouses or collect and harness data from different sources manually.
Common applications
Since data warehouse can be an invaluable asset for business users to back up this or that decision, it comes to serves domains that heavily rely on feedback systems for enterprise management, such as the following:
- Banking and finance
- Manufacturing and distribution
- Healthcare
- Insurance
- Retail
Introducing the data lakehouse
Data lakehouse is an alternative take on traditional storage solutions that unites the best of both worlds, the benefits of a data lake and a data warehouse — hence, the name. The lakehouse architecture has the scale, volume, and flexibility of a lake and the structure of a warehouse. It is to provide users with immediate access to a more simplified and more intuitive data repository, as it brings the additional metadata layer, and allows teams to access the enterprise data of multiple projects through a single system. Except for being a low-cost solution, in a lakehouse, the data can also be optimized for SQL performance — another reason to implement a lakehouse.
Main takeaways
In the age of big data, the evolving variation of emerging terms such as lakes, swamps, warehouses are not deprived of confusion or misuse. Meanwhile, understanding their core differences and applications can streamline your company’s data management, access, and prospective usage, giving you a considerable edge in the long run. Now, how do you store your data? We hope this article provided you with the necessary background to help you choose the best repository for your particular case.
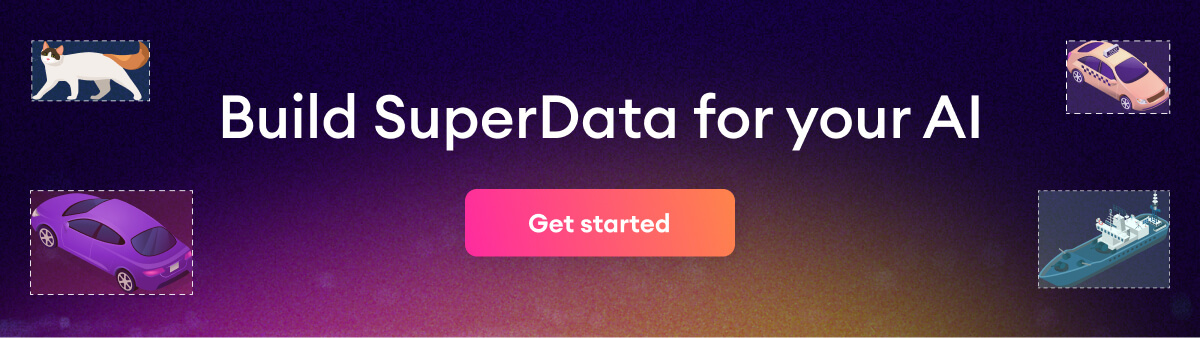