TACK project overview
Civil infrastructure such as bridges and tunnels are directly related to the overall economic and demographic growth of countries all over the world. The majority of these infrastructures are becoming older and they are increasingly prone to catastrophic failures that result in loss of lives and high costs. To prevent infrastructure damage and failure, stringent safety regulations have been recently defined. For this reason, more efficient monitoring systems are required by road administrators to determine the health and safety level of tunnels, bridges, and other infrastructures.
In general, cracks are associated with concrete degradation and reinforcement corrosion which reduce the infrastructural capacity. Cracks could, therefore, lead to a failure of infrastructures; for this reason, they should be detected as soon as possible and measured during the inspections that are routinely performed over time. In the latest years, infrastructure monitoring and maintenance has been performed by in-situ surveys that are expensive and time-consuming.
Recently, inspections have also been carried out by using a semi-automatic method where a mobile mapping equipment mounted on a vehicle is employed to acquire the scene and to reconstruct the 3D model of the infrastructure using a set of geomatics sensors (i.e., visible and infrared cameras, laser scanning, IMU). In particular, this digital representation, the so-called “digital twin”, of the infrastructure is subsequently analyzed manually by visual inspection for finding cracks and marking their extent. However, due to a large amount of collected data, this approach is still time-consuming, inefficient, and affected by errors.
TACK is an ongoing research project carried out by KTH - Royal Institute of Technology, Sapienza University of Rome, and WSP Sweden under the InfraSweden2030 program funded by Vinnova and Trafikverket [1]. TACK is supported by SuperAnnotate and KTH Innovation and it has also been recently selected by the Royal Swedish Academy of Engineering Sciences as one of the top 100 innovative research projects (IVA's 100 list 2020).
TACK aims at the development of a methodology for high-efficient and accurate monitoring of infrastructures using mobile mapping systems for data acquisition. The aim of this research and development project is to investigate and develop a new technique to detect cracks affecting tunnels and bridges using a hybrid approach of deep learning (Convolutional Neural Network – CNNs) and photogrammetry. This will give detailed information regarding the condition of the whole infrastructure which then will be used to assess its level of safety. With the developed technique, cracks will be automatically detected and measured from the imagery acquired using a customized mobile mapping system which leads to a highly efficient infrastructure monitoring and maintenance that can increase the safety of the infrastructures. An overview of the methodology of the TACK project is shown in Figure 1.
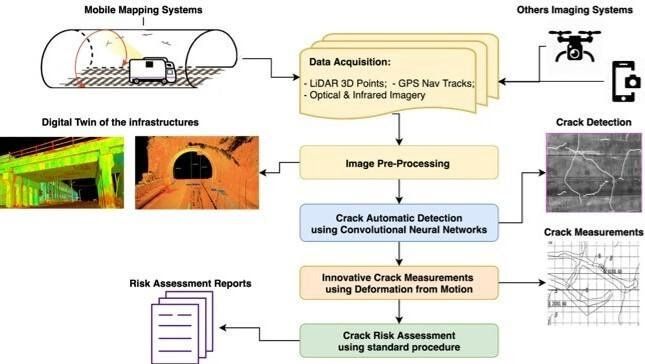
Through the development of new technology to automatically detect and monitor the propagation of cracks in tunnels and bridges, this project is in line with InfraSweden 2030 goals to create sustainable infrastructure. The main innovative aspect and challenge of the project is related to the integration of advanced deep learning approaches with an innovative photogrammetric algorithm developed in the recently finalized SBUF-founded project “Utvärdering av fältmetod för mätningar på sprutbetong”. The aim of the project is to raise the overall TRL (Technology Readiness Level) of the proposed system from the TRL 3-4 (TRL 3 – experimental proof of concept; TRL 4 – technology validated in lab) to a TRL 7 – system prototype demonstration in an operational environment.
Image annotation using SuperAnnotate
In the project, U-Net based neural networks are investigated to detect cracks in the images acquired using mobile mapping systems. To train CNN networks, a set of labeled images is always necessary. However, the procedure of data labeling is really time-consuming and it represents a crucial part since the way cracks are labeled highly affects the performances of the trained model.
During the first phase of the project, a preliminary test has been performed to train a CNN model using labeled images from an available online dataset [2]. The obtained results are promising. However, to improve the performances of the network and cover unexpected real-world situations, more photos including images of tunnels and bridges need to be adopted to train the model. For this reason, hundreds of images with cracks were selected from our tunnel and bridge datasets and other available online datasets [3] to perform crack annotation.
To speed up the procedure of image annotation, SuperAnnotate was adopted. The online platform represents a very powerful instrument for image annotation since it allows people to work together on the same project and check the quality of the annotations. Moreover, among the tools it provides, the “Smart Segmentation” option can guide annotators during the labeling to speed up the process. Two examples of “Smart Segmentation” applied to crack detection are presented in Figure 2. It is worth noticing that the tool can partially detect the shape of the crack and reduce the work of annotators.
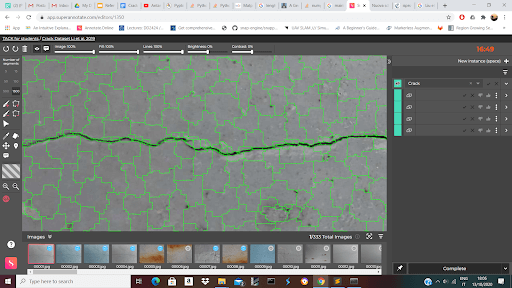
Finally, some of the images of the available datasets labeled using SuperAnnotate are shown in Figure 3, 4, and 5.
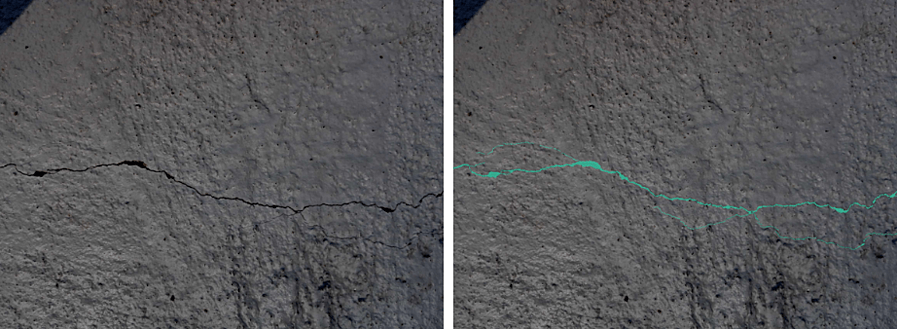
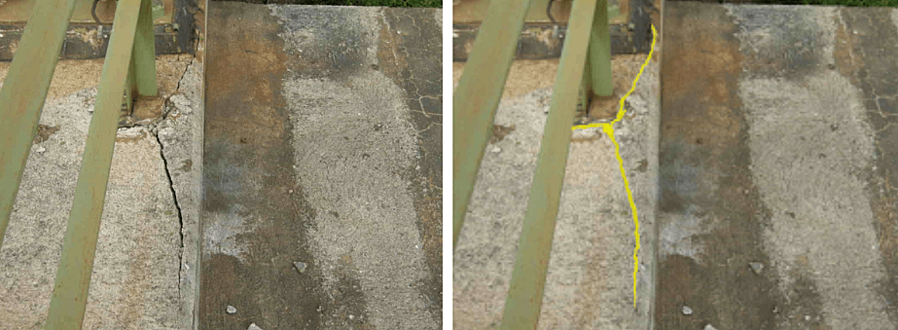
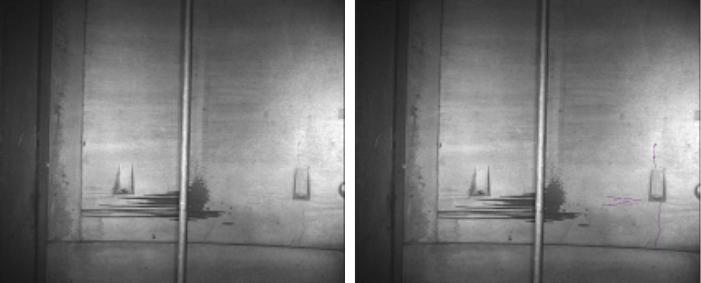
Authors
Valeria Belloni, PhD student at Sapienza University of Rome, Italy
Andrea Nascetti, Research scientist at KTH Royal Institute of Technology and Associate Professor at Politecnico di Bari, Italy
Andreas Sven Sjölander, Postdoc at KTH Royal Institute of Technology
References
[1] https://www.tackproject.xyz/
[2] Belloni, V., Sjölander, A., Ravanelli, R., Crespi, M., and Nascetti, A.: TACK PROJECT: TUNNEL AND BRIDGE AUTOMATIC CRACK MONITORING USING DEEP LEARNING AND PHOTOGRAMMETRY, Int. Arch. Photogramm. Remote Sens. Spatial Inf. Sci., XLIII-B4-2020, 741–745, https://doi.org/10.5194/isprs-archives-XLIII-B4-2020-741-2020, 2020
[3] Li, Shengyuan and Zhao, Xuefeng,: Image-Based Concrete Crack Detection Using Convolutional Neural Network and Exhaustive Search Technique, Advances in Civil Engineering, 1-12. 10.1155/2019/6520620, 2019