Some practice sports to maintain health and mindfulness, while others enjoy watching matches with friends. Regardless of our lifestyles and preferences, sports are definitely an integral part of our reality. Like any other significant realm of our daily lives and world economy, sport is inevitably subject to technological advancements.
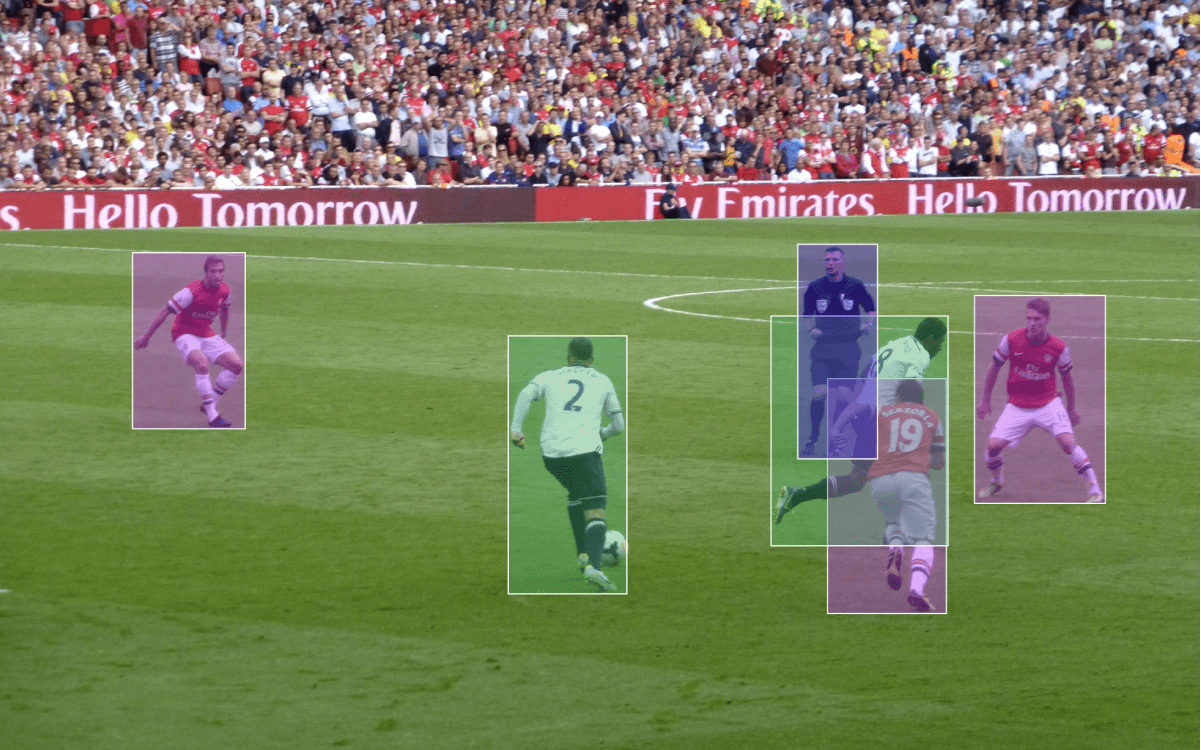
Today, in 2022, the real-time football analytics or sensor-equipped F1 cars are no longer faraway tech fantasies. In fact, the progress goes much beyond this: the most developed companies have already employed artificial intelligence and computer vision applications in sports, tackling various challenges. Given the great impact that technology has brought into sports, there is no doubt that artificial intelligence and machine learning will continue to push this field forward.
In this article, we will particularly focus on the role of computer vision in sports through the following breakdown:
- Computer vision in a nutshell
- Applications of computer vision in sports
- Challenges and limitations
- Sports datasets
- Key takeaways
Computer vision in a nutshell
Computer vision is the ability of computers or machines to process visual information such as digital images, videos, and more. We at SuperAnnotate have compiled several articles to give you a headstart if you're new to the industry:
- Introduction to computer vision: History and applications
- Top 3 trends in computer vision for 2023
- Machine learning and computer vision terms: All you need to know
- Top 15 computer vision libraries
- Top 15 must-read computer vision books
- Best computer vision courses for 2023
Without further ado, let's jump into the use cases of computer vision applications in sports.
Applications of computer vision in sports
Though fairly new, the terms artificial intelligence and deep learning are already transforming a number of essential industries, including healthcare, security, and of course, sports.
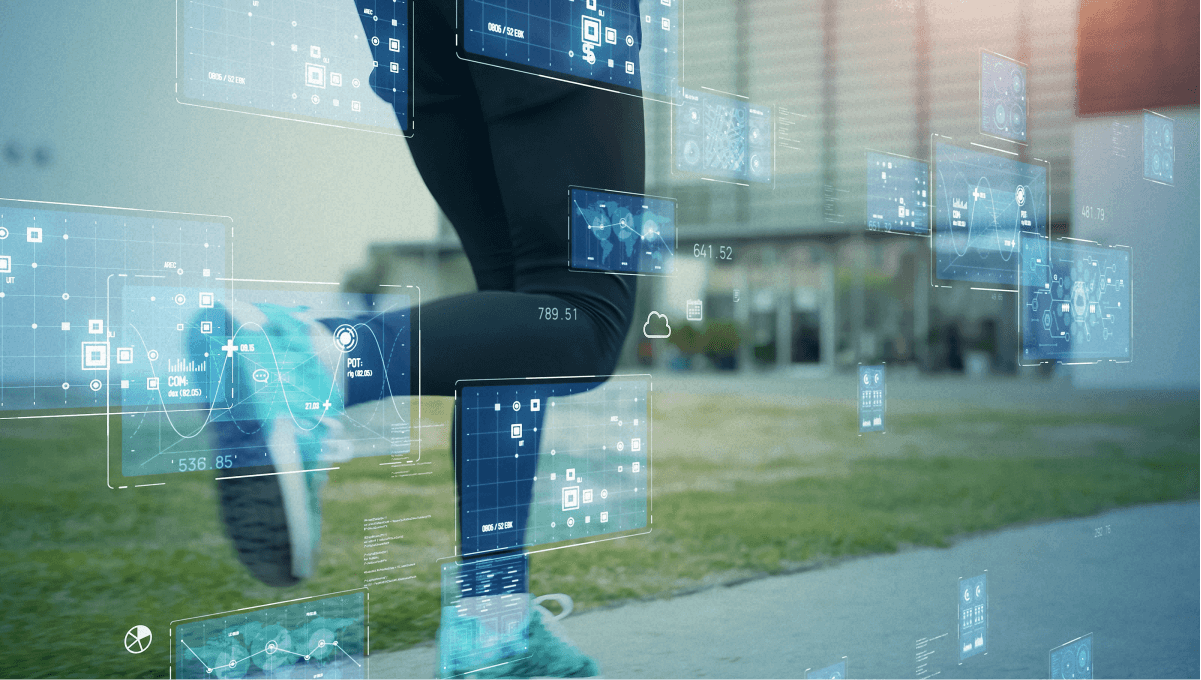
Most major sports involve fast and swift motion that is hard to follow and perceive with the naked eye. The ability to follow this motion is the first and most important application of machine learning in sports. Besides, computer vision technology in sports help process vast amounts of visual data received throughout the games to make match-related decisions in real-time, develop new training schemes, and much more. These computer vision solutions are of extreme help in data collection, sports analytics, and prognosis. Below are some of the recent applications of computer vision in sports:
Player tracking
Player tracking is one of the most popular tasks of computer vision sports. This involves detecting a single player or following several players at once with bounding boxes or key-point annotations (skeletons). Why are coaches or performance analysts interested in tracking player and team performance? The main reason is that it allows us to analyze how individual players move throughout the game and detect patterns in their behavior.
Not only can a computer vision system detect and track sports teams' players, but it can also generate semantic information. Machine learning can create context on players' actions and see, assume, or predict if the player has the ball, whether he/she passes, runs, defends, etc.
This use of technology in sports provides another possible advantage – a computer vision-powered system can suggest optimal player positions and display a comparison with actual positions in that specific game. In that way, players will clearly see the areas of growth and coaches will be able to better analyze players' performance.
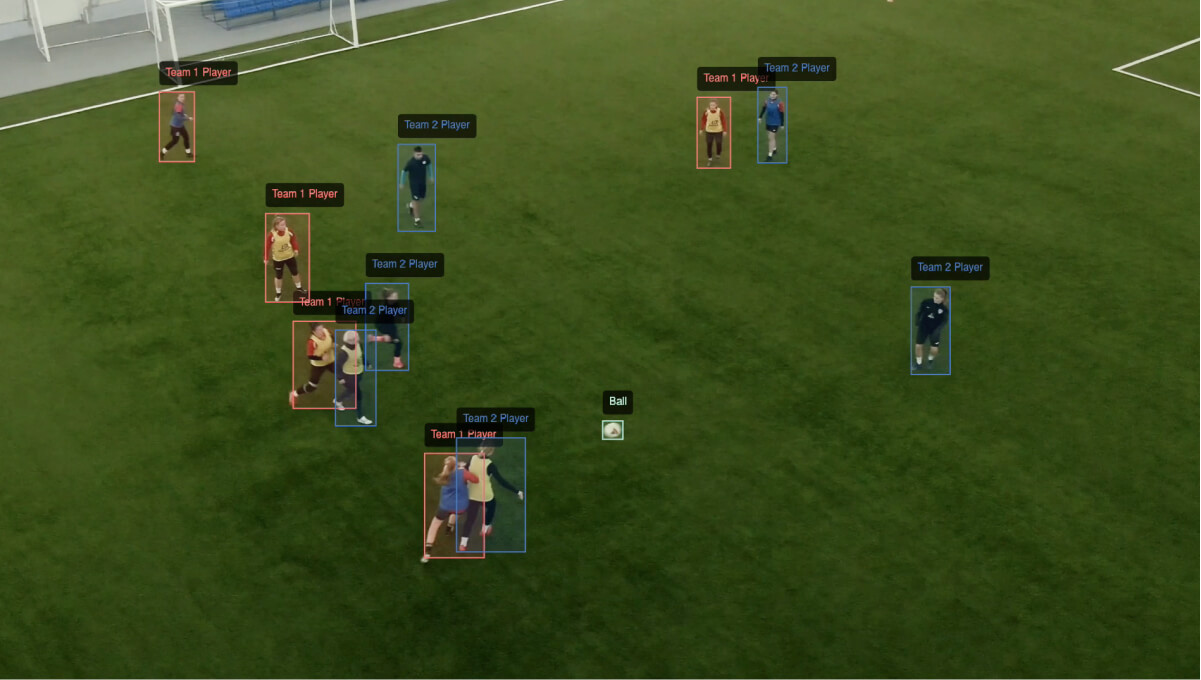
Ball tracking
Tracking ball movement is important for extracting information from ball-based sports, especially racket or bat-and-ball sports such as tennis, cricket, badminton, and more. Computer vision models can help record the ball's movement in three dimensions, show exactly where a ball hit the ground, and even predict its future trajectory to determine whether it would have hit the wicket.
In other words, computer-vision-powered ball tracking systems assist in:
- Ball detection
- Trajectory tracing
- Game results prediction
When it comes to specific sports such as basketball, volleyball, and soccer, this kind of ball tracking is more complicated because the ball can be hidden from view behind the players. Or alternatively, players' interactions with the ball can be rapid and unpredictable.
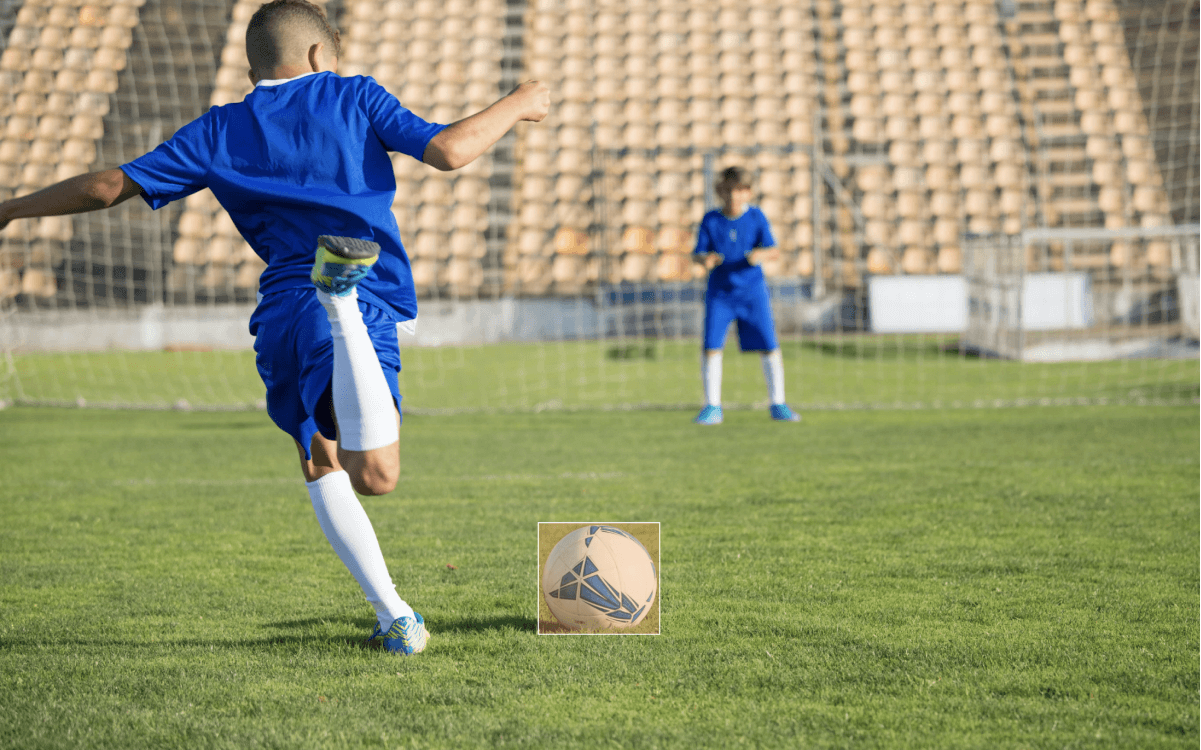
Injury prevention
Many people are turning to virtual classes to meet the growing need for mental rewiring and well-being with social distancing around. For example, both pilates and yoga are easy enough to do at home, however — especially for a beginner — it is important to try a class or two taught by a seasoned instructor, in a private or group setting, to learn how to exercise safely and avoid injuries. That's where computer vision, particularly pose estimation, steps in. Pose estimation is a computer vision task aimed at predicting and tracking the location of a person or object and 3D pose estimation-based apps are here to assist human fitness trainers. Using abundant motion tracking data, these technologies can analyze every movement of the user and provide them with detailed live feedback. This type of collaboration with a virtual coach helps receive real-time feedback and prevent injuries when exercising.

Enhancing viewer experience
Sports computer vision has benefits outside the field and training rooms, as it can even improve the whole process also for fans. As they know where and when to focus, computer-vision-powered cameras can automatically offer scenes from the action instead of providing a panoramic scene of the entire court. These same cameras can also be used to monitor and analyze fan reactions during games, which in turn helps in building fan engagement statistics.
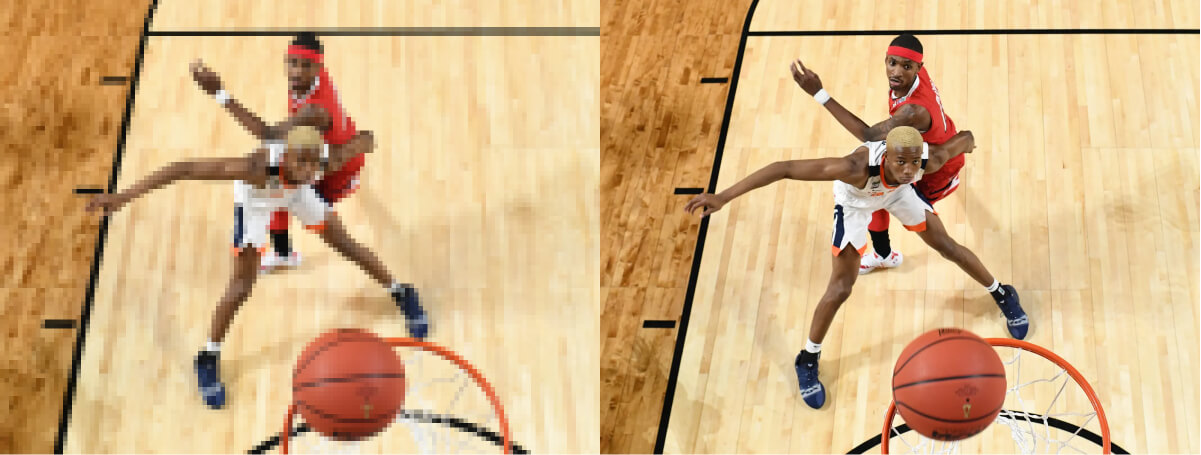
Better training sessions
Computer vision is needed to analyze player performance, whereas object recognition software tracks athletes and highlights the flaws in their techniques. With such advanced statistical analysis, players can not only learn from their own mistakes but also from their opponents.

Challenges and limitations
Computer vision in sports heavily depends on camera systems to obtain and later process sports footage. Typically, several cameras are placed close to the location where the event takes place, like the sidelines of a training field or the stands in a stadium during a match. The angle, positioning, hardware, and other shooting setups are different for each sport, and even within the same match. This poses a certain challenge for computer vision systems because they also need to be adjusted and tailored to specific matches and footage acquisition styles. A few more challenges include:
- Advanced filming equipment is unavailable for many sports clubs and performance analysis departments.
- Broadcast cameras often change their pan, tilt, and zoom which presents additional challenges for computer vision video processing systems to adjust to the dynamically changing data they receive.
- In certain circumstances, it may be challenging for computer vision systems that process videos to differentiate between the background and players, players and objects, players with the same outfit, and more.
However, computer vision has addressed these shortcomings to some measure. For example, computers are now able to distinguish between the ground, players, and other foreground objects due to image processing. Also, color-based segmentation algorithms can detect the grass by its green color, facilitating pitch zone detection, tracking moving players, and identifying the ball.
Sports datasets
For those interested in digging further into the topic and experimenting with computer vision in the sports industry, here is a list of ready-made public datasets.
1. Yoga pose image classification dataset
The dataset contains a total of 5994 files divided among 107 directories (folders), each representing a distinct yoga type. This dataset can help solve pose estimation tasks in yoga applications.

2. OpenTTGames dataset
OpenTTGames is a public dataset with five training and seven testing videos for computer vision tasks in table tennis. Each video includes ball coordinates markup files, a folder with segmentation masks, and a total of 4271 manually annotated events of 3 classes - ball bounces, net hits, and empty events.

3. NBA SportVU
The NBA SportVU dataset is publicly available on GitHub. It contains player and ball trajectories for 631 games from the 2015-2016 NBA season. The tracking data is in JSON format, and each moment includes information about the identities of the players on the court, the identities of teams, the period, the game clock, and the shot clock.
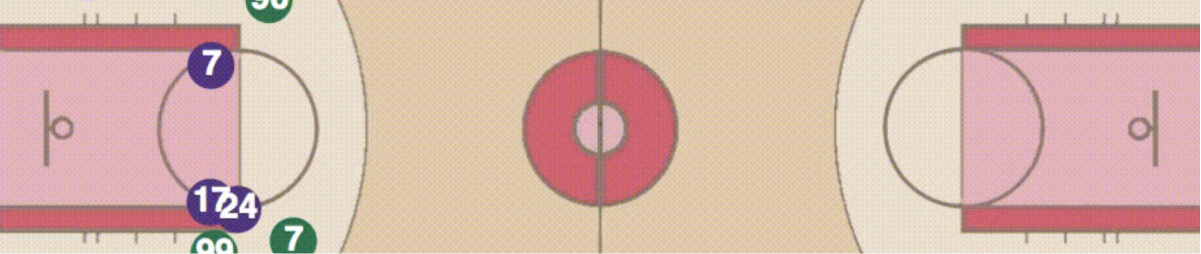
4. PoseTrack
PoseTrack is an open-source dataset for human pose estimation and articulated tracking in video. With both training and test sets, PoseTrack covers the following:
- 1356 video sequences
- 46K annotated video frames
- 276K body pose annotations
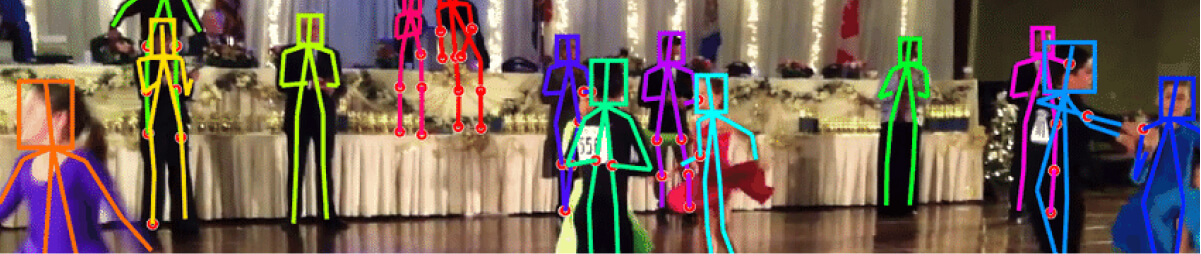
5. KTH Multiview Football Dataset II
Open for academic research, the KTH Multiview Football Dataset II consists of two major sets with 3D and 2D ground-truth pose estimation data. The 3D set alone includes 800-time frames, captured from 3 views (2400 images), 2 different players, and two sequences per player with 14 annotated joints.
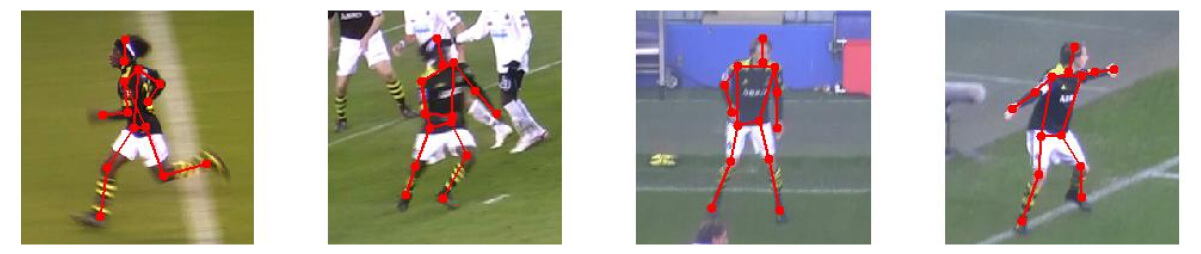
Key takeaways
Artificial intelligence is finding its way into all sorts of different sports, from baseball to football and even golf. In this article, we touched upon some of the most common use cases of the computer vision model in sports and illustrated examples of existing applications. The most popular sports computer vision tasks include player and ball tracking, pose estimation for injury prevention, segmentation for differentiating the background from players, and more.
Because computer vision is all about how you process visual data, we suggest you take advantage of public sports datasets and experiment with your projects. For more elaborate projects crafting your own image or video datasets is necessary, and that's where SuperAnnotate can help you build ground-truth data for your AI.
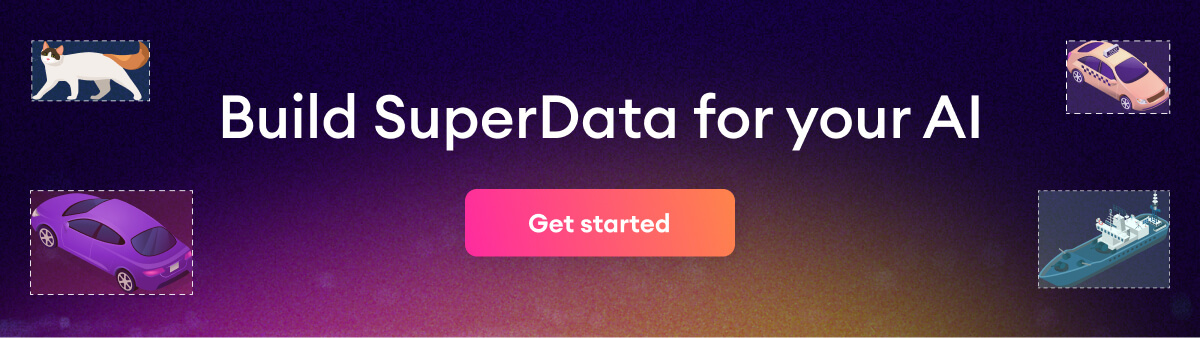