Artificial intelligence (AI) is advancing increasingly rapidly, continuing to drive innovation and making its presence felt across almost every industry. With the rising AI adoption, it comes as no surprise that the global artificial intelligence market size is expected to increase dramatically and reach $1394.30 billion by 2029, growing at a CAGR of 20.1% during the forecast period of 2022-2029, according to Fortune Business Insights.
As the AI industry is evolving and becoming more prevalent, new exciting trends constantly emerge to accelerate digital transformation and enable companies to adapt their digital product strategy to global business challenges. In this article, we’ll look into some major artificial intelligence developments in 2022 and explore the most anticipated AI trends in 2023.
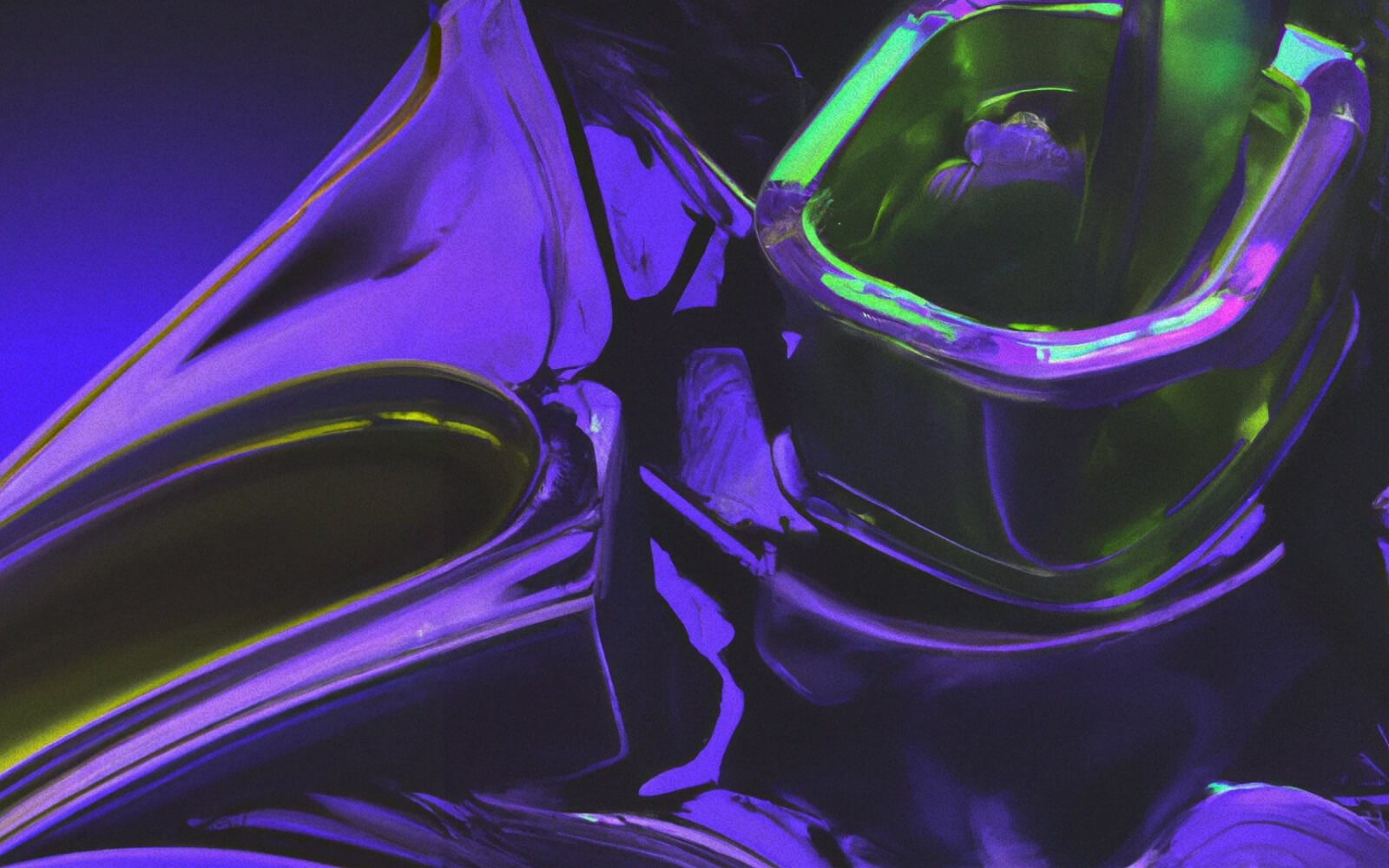
Revisiting 2022 AI trends
Below are the most common AI trends everyone has been talking about in 2022:
MLOps
MLOps has definitely been one of the biggest AI trends in 2022, with more companies and platforms leveraging it to increase the pace of model development and production. According to a 2021 McKinsey report, MLOps is a distinguishing factor among advanced artificial intelligence companies vs. non-practitioners.
Data-centric AI
Data-centric AI has also been gaining momentum as one of the top AI trends projected to trigger innovation in two to five years, as the 2022 Gartner Hype Cycle states. With the increase of data available today, data quality becomes increasingly crucial in determining the performance of an artificial intelligence model. Data-centric AI shifts the focus from model development to data quality needed to build more accurate and successful machine learning applications.
Generative AI
Generative AI is another evolving AI trend that has been disrupting many industries. With the significant progress in algorithms, Generatie AI can now create unique content like images, video, music, text, etc. According to Gartner, Generative AI is a strategic technology that will account for 10% of all data generated by 2025.
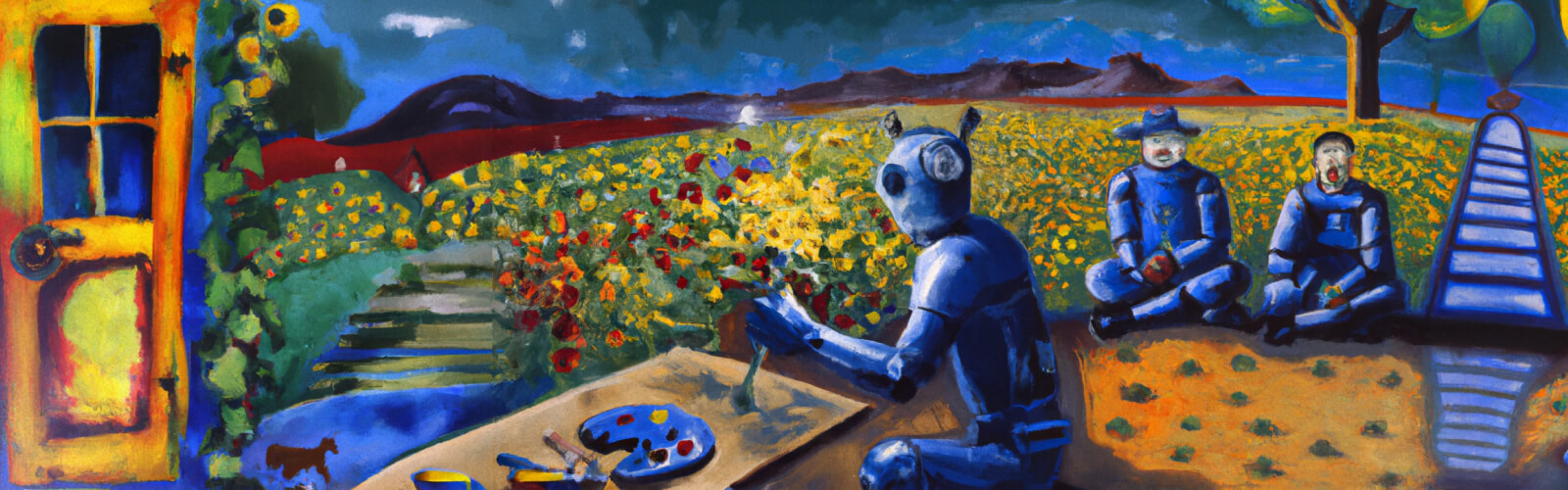
Along with Generative Adversarial Networks (GANs) that have been long around, this year, there has also been a lot of hype about text-to-image, image-to-image, image-to-video, and other varieties of generative algorithms, including Dall-E, ChatGPT, Stable Diffusion, that have been revolutionizing different benchmarks. Among other generative AI tools that have become a big blast this year are also powerful image and text embedding model CLIP released by Open.AI, BLIP, Stable Diffusion 1 and 2, eDiff-I, which are all ground-breaking generative AI algorithms that help ML engineers in handling different tasks.
Large language models
As large language models grow, they become more refined in language understanding and produce more human-like interactions. Large-scale models such as BERT and GPT-3 have been triggering a lot of hype for a while now, still, we have seen a lot more this year as these models have become a milestone in the field of artificial intelligence, bringing remarkable success to many niche NLP applications. With sophisticated pre-training objectives and a large number of model parameters, large language models can effectively extract knowledge from a vast amount of labeled and unlabeled data. They have also proven to generalize quite well with zero-shot and few-shot learning for new tasks.
Along with the rapid progress in large language models showing no signs of slowing down, new breakthrough models have come out, including NLLB, BLOOM, and Copilot/Codex.
NLLB, coined as No Language Left Behind, introduces an automatic dataset construction approach to improve low-resource language coverage. NLLB-200 translates across 200 languages and is built with a Sparse Mixture-of-Experts model to leverage shared capacity and significantly improve the translation for low-resource languages. Regularization techniques, large-scale data augmentation, and self-supervised learning are also used. In addition, the human-verified evaluation benchmark, FLORES-101, is extended twice to include more languages and compare with other translation models for improving the evaluation of translation quality across more languages.
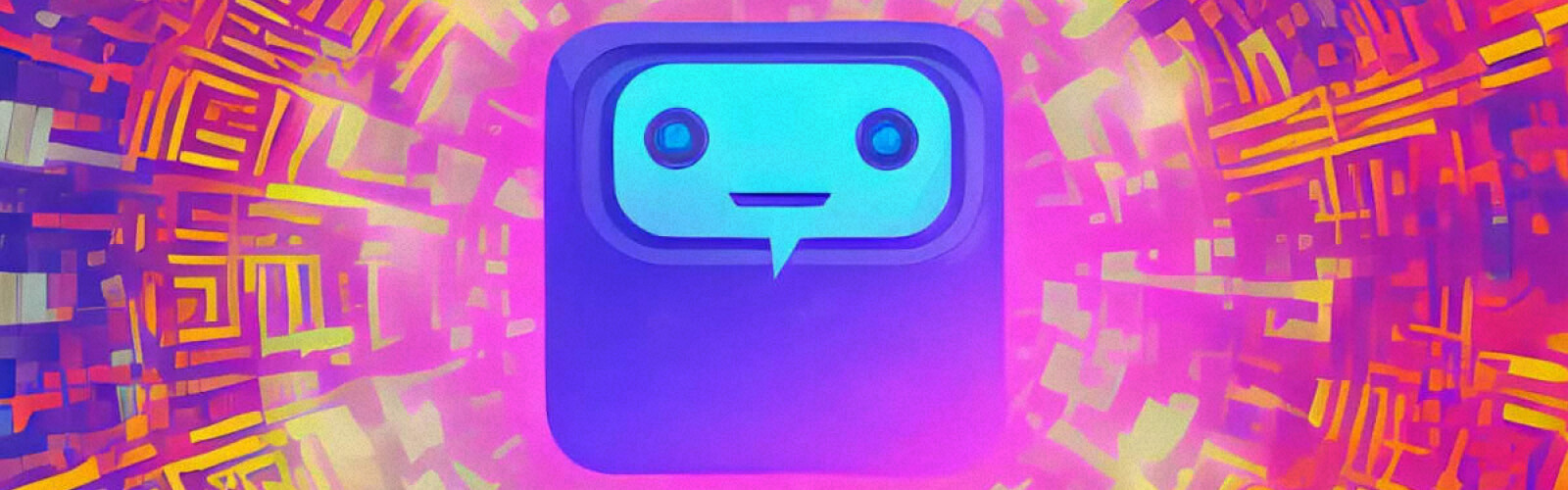
BLOOM, an open-access multilingual language model, is another alternative to GPT-3 with 176 billion parameters. The model has been trained on vast amounts of textual data sources, including scientific articles, literature, sports news, and can generate coherent text in 46 languages and 13 programming languages.
Copilot is also emerging as a popular AI-powered coding tool that suggests whole lines of code and functions based on the context of the existing code. After Copilot's year-long technical preview, it has now become generally available with a subscription.
ChatGPT, an artificial intelligence chatbot system recently released by Open.AI, is another revolutionary AI technology that enables natural language processing (NLP) and interacts in a conversational way. Based on the InstructGPT model, ChatGPT comes with advanced capabilities and is basically trained with reinforcement learning models. To help "rate" the response from the ChatGPT model and further assist with training, OpenAI has trained a separate reinforcement learning model based on the PPO algorithm.
The latest release of the YoloV7 real-time object detection model for computer vision tasks is an important milestone in the advancement of real-time object detection models. YoloV7 comes with greater speed and accuracy than its previous versions and boasts many new and exciting features.
The expansion of transformers in the computer vision field is also noteworthy with LViT, for example, which extended vision transformers for the medical domain. Furthermore, Neural Radiance Fields involving interesting approaches to create 3D objects from 2D photos are also some of the most exciting developments.
Current challenges
These evolving artificial intelligence trends are exciting but they also bring challenges along the way. Some major issues remain in the Generative AI space in terms of bias and ethical concerns in training AI algorithms. Generative models do not always produce true content and do not always work best on creating texts, faces, or limbs and discerning colors of two or more objects, making it harder for humans to evaluate their truthfulness.
Lack of transparency in training data is another hurdle to overcome as many models are gated by companies, and no commercial access is available to the training data. Language compatibility is also a point of consideration, as most models are built on English, and there is little support for other languages. And above all, to effectively train and run AI models, businesses need large amounts of high-quality data which requires significant resources and generates high costs.
Looking ahead to AI trends in 2023
Now that we have covered the biggest artificial intelligence trends of the year let's look to the future and explore some of the most promising AI trends for 2023.
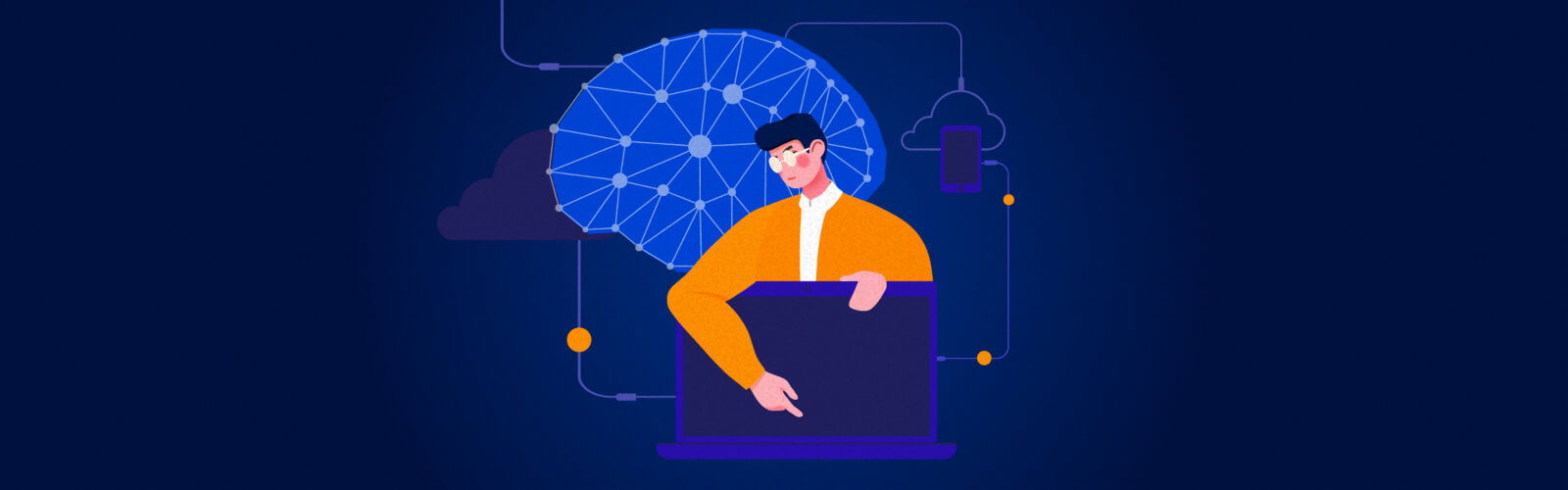
Multimodal models
Multimodal models incorporate several modalities, say text, images, audio, and video at the same time to extract features from all sources and produce more robust predictions at a larger scale. Multimodal systems analyze different types of information, giving an AI system a wider understanding of the task and expanding its capabilities.
The approach of training models on different data types also helps increase the accuracy of an AI model. Multimodal data are semantically correlated and can combine audio, visual, text, or other available inputs to ensure a higher level of prediction accuracy.
Some interesting applications of multimodal models come from the automotive industry. For example, various techniques to combine Short-Range Radar, Long-Range Radar, LiDAR, Vision, and other modalities of data are used to train self-driving car models, while sensor fusion with artificial intelligence allows making sense of it all.
DeepMind’s Gato is another inspiration from large language models that works as a “generalist” AI model for various complex tasks. The deep learning transformer model enables feeding a lot of different inputs into a model, like text, images, text-image pairs, etc., all of which are parameterized similarly and flattened into a specific tokenized input to predict multimodal outputs.
Multimodal machine learning is a growing research field opening up many exciting possibilities that are already being embraced by the automotive, robotics, and healthcare sectors. The multimodal machine learning field has seen much progress recently, with big opportunities lying ahead. Many more promising applications are expected to drive a new wave of innovation in the near future.
Ethical and responsible AI
A study by McKinsey reveals that AI adoption has at least doubled between 2017 and 2022. While artificial intelligence technology is getting more embedded into the business landscape, it also generates ethical decision-making dilemmas. More organizations recognize the need for ethical and responsible AI to ensure their AI is trustworthy, reliable, and makes accurate and bias-aware decisions.
Ethics continues to be a very important topic for the development of AI applications because, if unregulated, AI can pose reputational, legal, and other risks. As large language models and generative space become more widespread, potential ethical issues of people using the AI models in different ways also arise. It is getting harder to regulate how people consume and engage with AI-generated content and how it is possible to restrict them from using this content for malicious purposes.
This year, for example, lots of discussions have been revolving around ethical concerns on releasing an API for Dall-E and the open-source release of Stable Diffusion, causing challenging ethical questions on their data usage.
Some common data bias issues also concern annotator bias, and other data labeling biases the system learns from. And while human intervention is still needed to ensure AI-supported decisions are fair, it can also be flawed as a result of unintentional individual and social biases.
So will fully automated decision-making be less biased and help humans make fairer decisions, or will it make it worse? While it’s still unclear what’s in the future for AI ethics, one thing is sure that minimizing bias in artificial intelligence is critical to unlock its full potential and increase people’s trust in AI systems. With that in mind, both ethical and responsible AI will be a leading priority for many AI applications in 2023.
Data privacy
Along with the accelerated advancement of digital technology, the world’s data doubles every two years, according to the Digital Universe Study conducted by IDC. Data is accumulated in real-time and at a rapid pace, with the most privacy-sensitive data analysis being driven by machine learning. As AI continues to evolve rapidly, it increases the potential for using personal data in ways that could generate privacy concerns.
Data privacy in AI remains a top priority for companies to ensure that the most accurate data is used to train the models and that datasets are viewed by the right people and with the right regulations. That’s something we can see in LLMs and generative models. For example, Google has not released the dataset for its model PaLM, which makes it impossible to verify and test the model, raising concerns about what the model can do and how it functions. Possible data privacy and bias concerns also come from the fact that these datasets have reportedly been trained on websites and social media conversations. The only thing users know is that these datasets have been trained on something like websites and social media conversations. So there might be a lot of leakage of sensitive information, and it might even be possible for personal information to leak into the models and become encoded.
Similarly, in many cases, users can extract personally identifiable information with queries, feed into large language models and access personal information that would not otherwise be available because of privacy concerns. Data privacy is definitely a space that will be much visible and talked about in the next few years.
Final thoughts
AI and machine learning technologies sit at the heart of the digital transformation and will be even more pervasive ahead. These technologies have come a long way in making smarter decisions, streamlining business processes, increasing productivity, and cutting costs.
We have explored the major trends shaping the artificial intelligence space in 2022 and will continue to see AI rising at scale in 2023 and beyond, unlocking new avenues for breakthrough innovations and never-before-seen applications.
Let us know your thoughts on the biggest artificial intelligence trends to watch in 2023, and we’ll cover relevant topics in our upcoming blog posts.